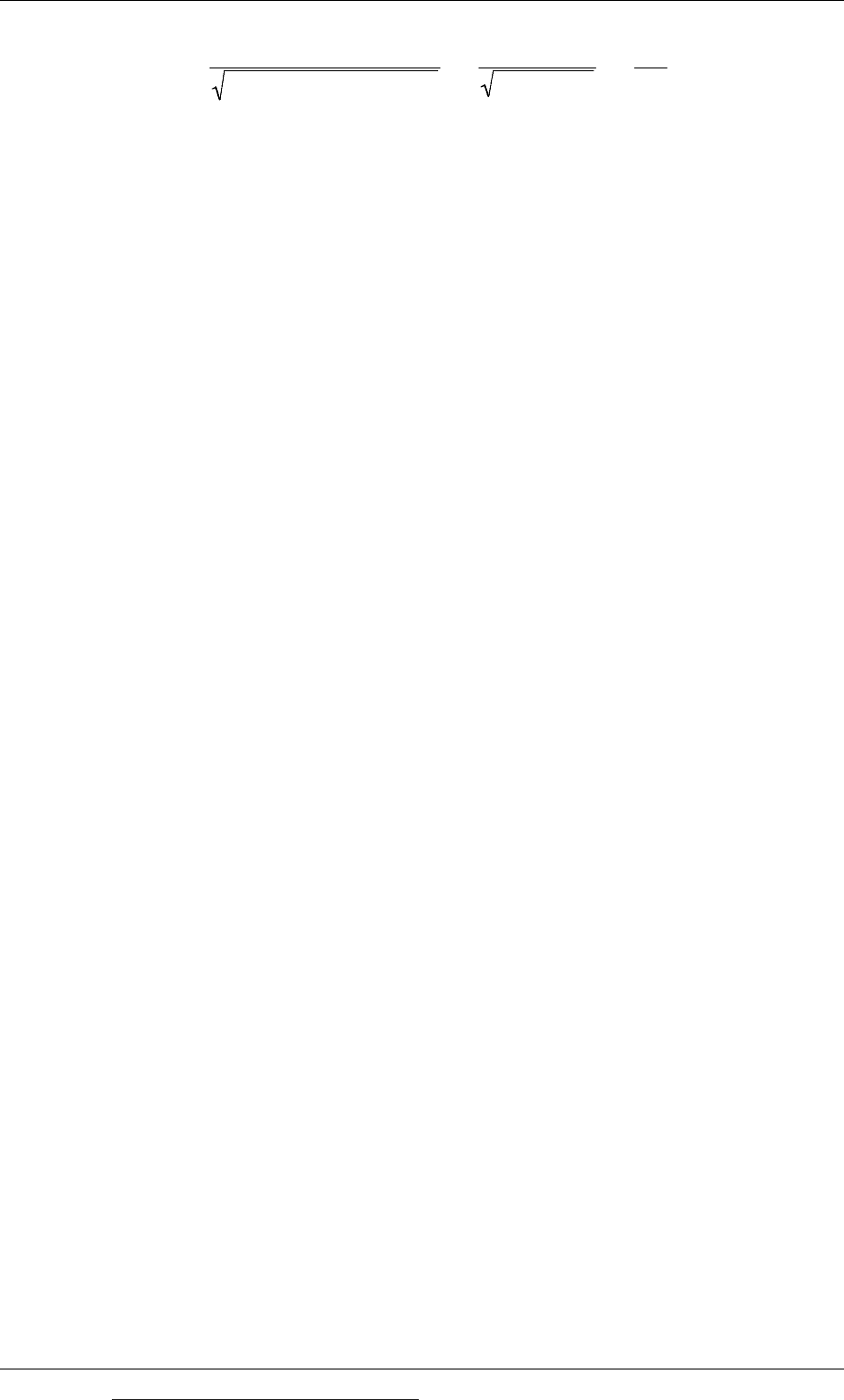
Paper F2: Management accounting
78 Go to www.emilewoolfpublishing.com for Q/As, Notes & Study Guides © EWP
r =
249
(41) [6(136,185) – (903)
2
]
=
249
(41) (1,701)
=
249
264
= +0.94
The correlation coefficient is + 0.94.
Significance of the correlation coefficient
The value of the correlation coefficient must always be in the range – 1 to + 1.
A value of – 1 indicates that there is perfect negative correlation between the
values for y and the values for x that have been used in the regression analysis
estimates. Perfect negative correlation means that all the values for x and y,
plotted on a graph, would lie on a straight downward-sloping line.
A value of + 1 indicates that there is perfect positive correlation between the
values for y and the values for x that have been used in the regression analysis
estimates. Perfect positive correlation means that all the values for x and y,
plotted on a graph, would lie on a straight upward-sloping line.
A value of r = 0 indicates no correlation at all between the values of x and y.
For cost estimation, a value for r close to + 1 would indicate that the cost estimates
are likely to be very reliable.
As a general guide, a value for r between + 0.90 and + 1 indicates good correlation
between the values of x and y, suggesting that the formula for costs can be used
with reasonable confidence for cost estimation.
If you calculate a value for r that is more than +1 or is a greater negative value than
– 1, your calculation will be wrong.
3.4 Coefficient of determination r
2
The square of the correlation coefficient, r
2
, is called the coefficient of determination.
The value of r
2
shows how much the variations in the value of y, in the data used to
calculate the regression analysis formula, can be explained by variations in the value
of x.
Significance of coefficient of determination
The value of the coefficient of determination must always be in the range 0 to +1.
If the value of r is + 0.70, this means that on the basis of the data used in the
regression analysis formula, 0.49 or 49% (= 0.70
2
) of variations in the value of y
can be explained by variations in the value of x.
Similarly if the value of r is – 0.80, this means that on the basis of the data used
in the regression analysis formula, 0.64 or 64% (= 0.80
2
) of variations in the value
of y can be explained by variations in the value of x. Since r is negative, this
means that y falls in value as the value of x increases.
In the example above, where r = + 0.94, we can say that from the data used to
produce a formula for total costs, 88.36% (0.94 × 0.94 = 0.8836) of the variations in