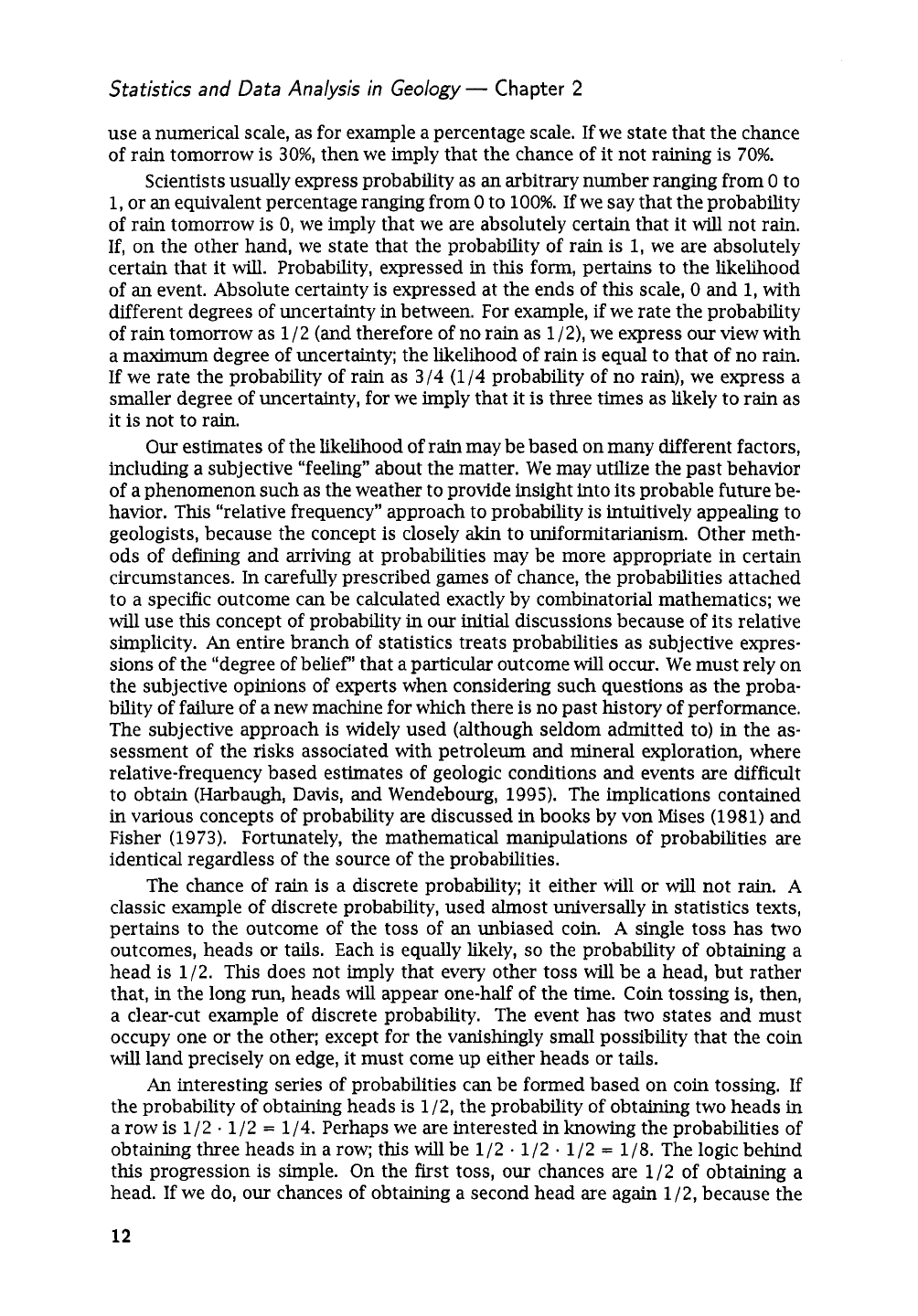
Statistics and Data Analysis in Geology
-
Chapter
2
use a numerical scale, as for example a percentage scale.
If
we state that the chance
of rain tomorrow is
30%,
then
we
imply that the chance of it not raining is
70%.
Scientists usually express probability as an arbitrary number ranging from
0
to
1,
or an equivalent percentage ranging from
0
to
100%.
If
we say that the probability
of
rain
tomorrow is
0,
we
imply that we
are
absolutely
certain
that it
will
not rain.
If,
on the other hand, we state that the probability of rain is
1,
we
are absolutely
certain that it
will.
Probability, expressed
in
this form, pertains to the likelihood
of
an event. Absolute certainty is expressed at the ends of this scale,
0
and
1,
with
different degrees of uncertainty
in
between. For example, if we rate the probability
of
rain
tomorrow as
1
/2
(and therefore
of
no rain as
1
/2),
we express our view with
a maximum degree of uncertainty; the likelihood of rain is equal to that of no rain.
If
we rate the probability of rain as
3/4 (1/4
probability of no rain), we express a
smaller degree of uncertainty, for we imply that it is three times as likely to rain as
it is not to rain.
Our estimates of the likelihood of rain may be based on
many
different factors,
including a subjective “feeling” about the matter.
We
may utilize the past behavior
of a phenomenon such as the weather to provide insight into its probable future be-
havior. This “relative frequency” approach to probability is intuitively appealing to
geologists, because the concept is closely
akin
to uniformitarianism. Other meth-
ods
of defining and arriving at probabilities may be more appropriate in certain
circumstances. In
carefully
prescribed games of chance, the probabilities attached
to a specific outcome can be calculated exactly by combinatorial mathematics; we
will use this concept of probability
in
our initial discussions because of its relative
simplicity.
An
entire branch of statistics treats probabilities as subjective expres-
sions of the “degree of belief” that a particular outcome will occur.
We
must rely on
the subjective opinions of experts when considering such questions as the proba-
bility of failure of
a
new machine for which there is no past history of performance.
The subjective approach is widely used (although seldom admitted to) in the as-
sessment
of
the risks associated with petroleum and mineral exploration, where
relative-frequency based estimates of geologic conditions and events
are
difficult
to obtain (Harbaugh, Davis, and Wendebourg,
1995).
The implications contained
in various concepts of probability
are
discussed in books by von Mises
(1981)
and
Fisher
(1973).
Fortunately, the mathematical manipulations of probabilities are
identical regardless of the source of the probabilities.
The chance of rain is a discrete probability;
it
either will or
will
not rain.
A
classic example of discrete probability, used almost universally
in
statistics texts,
pertains to the outcome of the toss of an unbiased coin.
A
single toss has two
outcomes, heads or tails. Each is equally likely,
so
the probability of obtaining a
head is
1/2.
This does not imply that every other toss will be a head, but rather
that,
in
the long
run,
heads will appear one-half of the time. Coin tossing is, then,
a clear-cut example
of
discrete probability. The event has two states and must
occupy one or the other; except for the vanishingly small possibility that the coin
will land precisely on edge, it must come up either heads or tails.
An
interesting series of probabilities can be formed based on coin tossing.
If
the probability of obtaining heads is
1/2,
the probability of obtaining two heads in
a row is
1/2
.
1/2
=
1/4.
Perhaps we are interested in knowing the probabilities of
obtaining
three
heads in
a
row; this will be
1/2
.
1/2
-
1/2
=
1/8.
The logic behind
this
progression is simple.
On
the first toss, our chances
are
1/2
of
obtaining a
head.
If
we do, our chances of obtaining a second head are again
1/2,
because the
12