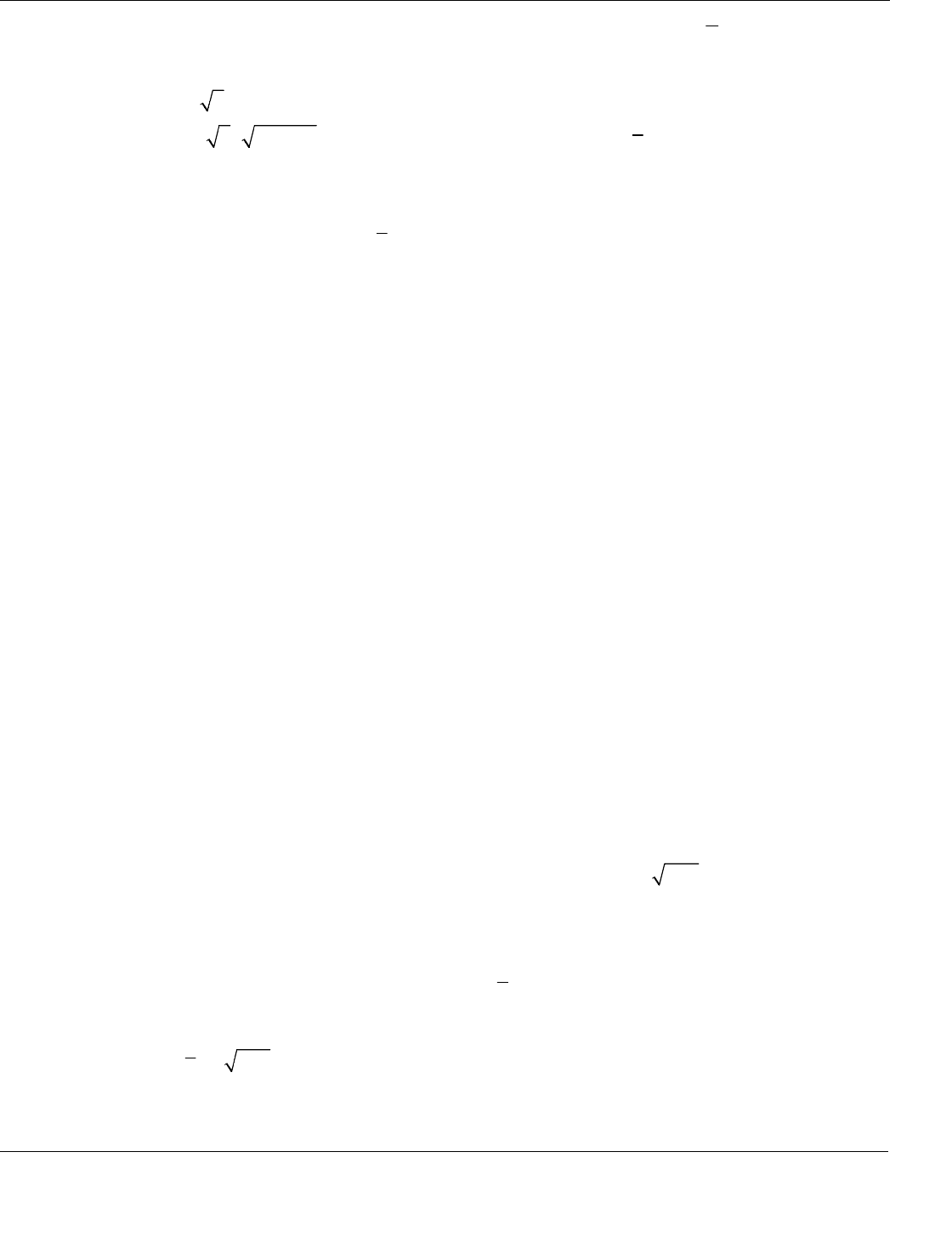
ptg6843605
sampling − sand cone model
Page 311 The Encyclopedia of Operations Management
Step 4. Recompute the sample mean and sample standard deviation – Recompute
and s from the entire
n observations.
Step 5. Compute the half-width and create the confidence interval – Compute the half-width
/2
' /h z s n
. If the sample size n is large relative to the total population N (i.e.,
/ 0.05n N
), use
/2
' / 1 /h z s n n N
instead. The confidence interval is then
'
h
.
Step 6. Check results – Make sure that
'h h
; if not, repeat steps 2 to 6.
The larger the number of observations (n), the smaller the confidence interval. The goal is to find the lowest
value of n that will create the desired confidence interval. If n observations are selected randomly many times
from the population, the confidence interval (
'
h
) will contain the true mean about 100(1 )%
of the time.
See central limit theorem, confidence interval, dollar unit sampling, sampling, standard deviation.
sampling – The selection of items from a population to help a decision maker make inferences about the population.
Sampling is frequently used when it is impossible, impractical, or too costly to evaluate every unit in the
population. Sampling allows decision makers to make inferences (statements) about the population from which
the sample is drawn. A random sample provides characteristics nearly identical to those of the population.
One major issue in developing a sampling plan is the determination of the number of observations in the
sample (the sample size) needed to achieve a desired confidence level and maximum allowable error. See the
sample size calculation entry for more details.
Probability sampling includes simple random sampling, systematic sampling, stratified sampling,
probability proportional to size sampling, and cluster or multistage sampling. Stratified sampling (also known
as stratification) defines groups or strata as independent subpopulations, conducts random samples in each of
these strata, and then uses information about the population to make statistical inferences about the overall
population. Stratified sampling has several advantages over simple random sampling. First, stratified sampling
makes it possible for researchers to draw inferences about groups that are particularly important. Second,
stratified sampling can significantly tighten the confidence interval on the mean and reduce the sample size to
achieve a predefined confidence interval. Finally, different sampling approaches can be applied to each stratum.
See acceptance sampling, Analysis of Variance (ANOVA), central limit theorem, confidence interval,
consumer’s risk, dollar unit sampling, hypergeometric distribution, Lot Tolerance Percent Defective (LTPD),
normal distribution, operating characteristic curve, producer’s risk, sample size calculation, sampling
distribution, standard deviation, t-test, work sampling.
sampling distribution – The probability distribution for a statistic, such as the sample mean, based on a set of
randomly selected units from a larger population; also called the finite-sample distribution.
A sampling distribution can be thought of as a relative frequency distribution from a number of samples
taken from a larger population. This relative frequency distribution approaches the sampling distribution as the
number of samples approaches infinity. For discrete (integer) variables, the heights of the distribution are
probabilities (also called the probability mass). For continuous variables, the intervals have a zero width, and the
height of the distribution at any point is called the probability density.
The standard deviation of the sampling distribution of the statistic is called the standard error. According
to the central limit theorem, the standard error for the sample mean is always / 1s n , where s is the standard
deviation for the sample. Other statistics, such as the sample median, sample maximum, sample minimum, and
the sample standard deviation, have different sampling distributions.
For example, an analyst is trying to develop a confidence internal on the average waiting time for a call
center where the waiting time follows an exponential distribution. The analyst collects n = 101 sample waiting
times and finds the sample mean and standard deviation are ( , )
s = (5.5, 1.2) minutes. According to the central
limit theorem, the sampling distribution for the sample mean is the normal distribution regardless of the
underlying distribution. The sampling distribution for the sample mean, therefore, is normal with mean and
standard deviation
( , / 1)x s n
= (5.5, 0.12) minutes with a 95% confidence interval of (5.26, 5.74) minutes.
See central limit theorem, confidence interval, probability density function, probability distribution,
probability mass function, sampling.