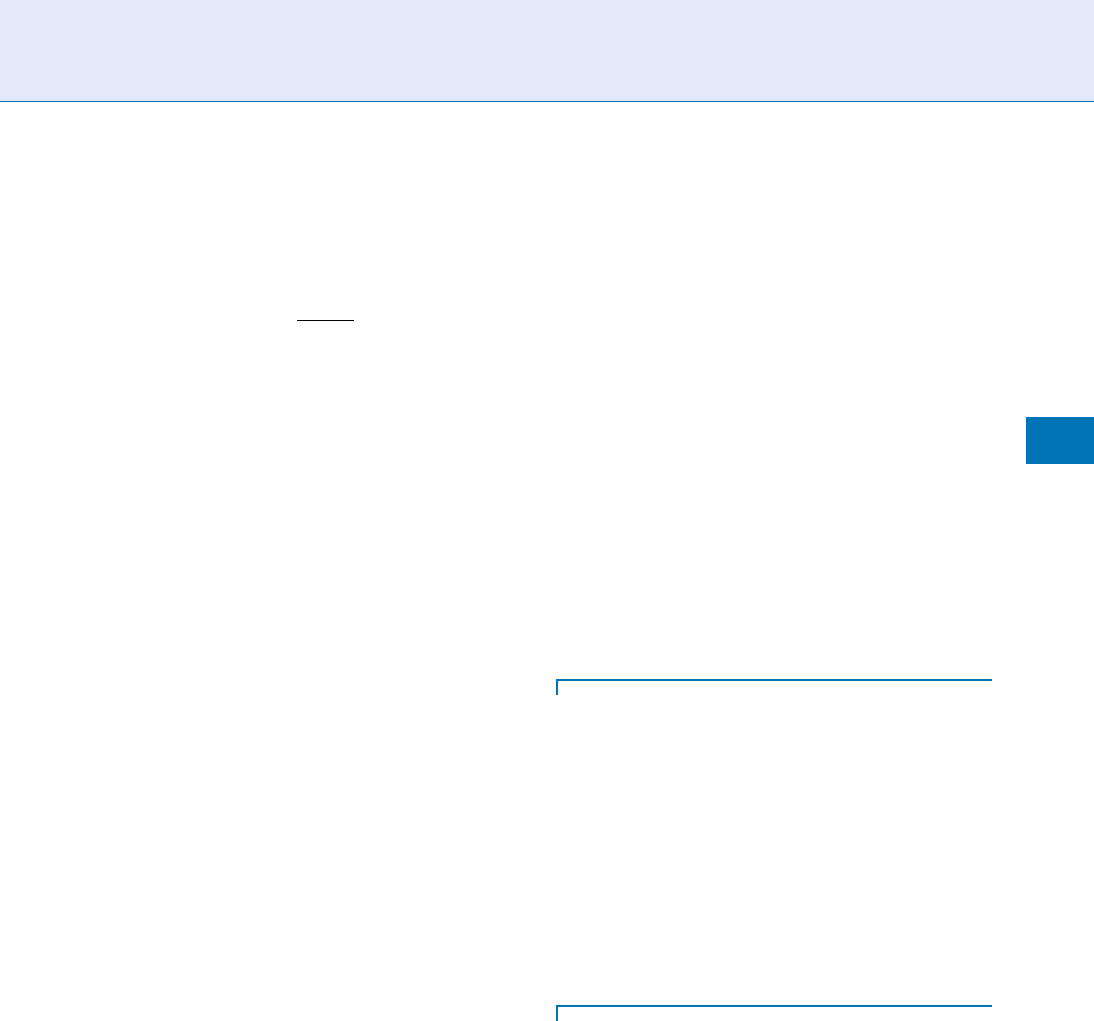
sufficient statistics, not the derived parameters, such as
the variance, are being adapted.
For each mixture and each parameter, a data-
dependent adaptation coefficient a
i
r
, r 2 {w, m, v},
is used in the equations mentioned earlie r. This is
defined as
a
r
i
¼
n
i
n
i
þ r
r
; ð15Þ
where r
r
is a fixed ‘‘relevance’’ factor for parameter r.
It is common in speaker recognition applications
to use one adaptation coefficient for all parameters
(a
i
w
¼ a
i
m
¼ a
i
v
¼ n
i
∕(n
i
þ r)) and further to only
adapt certain GMM parameters, such as only the mean
vectors.
Using a data-dependent adaptation coefficient
allows mixture-dependent adaptation of paramet ers.
If a mixture component has a low probabilistic count,
n
i
, of new data, then a
i
r
! 0 causing the de-emphasis
of the new (potentially under-trained) parameters and
the emphasis of the old (better trained) parameters.
For mixture components with high probabilistic
counts, a
i
r
! 1, causing the use of the new class-
dependent parameters. The relevance factor is a way
of controlling how much new data should be observed
in a mixture before the new parameters begin replacing
the old parameters. This approach should thus be
robust to limited training data.
Related Entries
▶ Session Effects on Speaker Modeling
▶ Speaker Matching
▶ Speaker Recognition, Overview
▶ Universal Background Models
Acknowledgment
This work was sponsored by the Department of
Defense under Air Force Contract FA8721-05-C-0002.
Opinions, interpretations, conclusions, and recom-
mendations are those of the authors and are not neces-
sarily endorsed by the United States Government.
References
1. Benesty, J., Sondhi, M., Huang, Y. (eds.): Springer Handbook of
Speech Processing, vol. XXXVI. Springer, Berlin (2008)
2. Mu
¨
ler, C. (ed.): Speaker Classification I: Fundamentals, Features,
and Methods, vol. 4343/2007. Springer: Lecture Notes in Com-
puter Science, Berlin (2007)
3. Gray, R.: Vector qantization. In: IEEE ASSP Magazine, pp. 4–29
(1984)
4. Reynolds, D.A.: A Gaussian Mixture Modeling Approach to
Text-Independent Speaker Identification. PhD thesis, Georgia
Institute of Technology (1992)
5. Reynolds, D.A., Rose, R.C.: Robust text-independent
speaker identification using Gaussian mixture speaker
models. IEEE Trans. Acoust. Speech Signal Process. 3(1), 72–83
(1995)
6. McLachlan, G. (ed.): Mixture Models. Marcel Dekker, New York,
NY (1988)
7. Dempster, A., Laird, N., Rubin, D.: Maximum likelihood from
incomplete data via the EM algorithm. J. Royal Stat. Soc. 39(1),
1–38 (1977)
8. Reynolds, D.A., Quatieri, T.F., Dunn, R.B.: Speaker verification
using adapted Gaussian mixture models. Digital Signal Process.
10(1), 19–41 (2000)
GC
GC is an analytical chemistry separation technique
which provides separation of mixtures on the basis of
differential affinity between a liquid or solid stationary
phase and a gas mobile phase.
▶ Odor Biometrics
Gelatin Pad
Gelatin lifting pads are designed for the lifting of
fingerprints, footprints, dust marks , and trace evi-
dences. They comprise three layers, the first layer is
the carri er, which holds the second layer of thick low-
adhesive gelatin in a pliable and flexible format. The
thick gelatin layer is ideal for lifting evidence without
sticking to the surrounding lift area. The third layer, a
cover sheet, is a clear polyester film which is removed
prior to lifting, and may be replaced once the lift is
completed.
▶ Footwear Recognition
Gelatin Pad
G
663
G