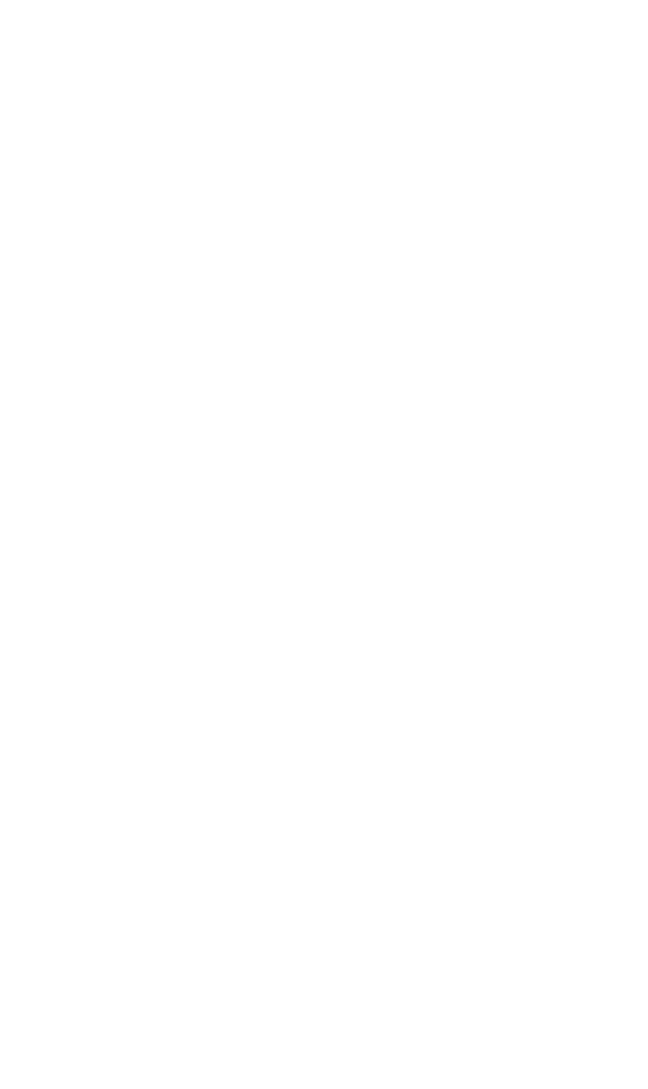
has a significant influence on the results. In order for all of the modal parameters to
be estimated, the subspace must encompass a region which includes contributions of
all three characteristics.An important example is the necessity to use multiple refer-
ence data (inputs and outputs) in order to estimate repeated roots. The particular
subspace which is measured and the weighting of the data within the subspace in an
algorithm are the main differences among the various modal identification proce-
dures which have been developed.
In general, the amount of information in a measured subspace greatly exceeds
the amount necessary to solve for the unknown modal characteristics. Another
major difference among the various modal parameter estimation procedures is the
type of condensation algorithms that are used to reduce the data to match the num-
ber of unknowns [for example, least squares (LS), singular value decomposition
(SVD), etc.]. As is the case with any overspecified solution procedure, there is no
unique answer.The answer that is obtained depends upon the data that are selected,
the weighting of the data, and the unique algorithm used in the solution process. As
a result, the answer is the best answer depending upon the objective functions asso-
ciated with the algorithm being used. Historically, this point has created some con-
fusion since many users expect different methods to give exactly the same answer.
Many modal parameter estimation methods use information (subspace) where
only one or two characteristics are included. For example, the simplest (computa-
tionally) modal parameter estimation algorithms utilize one impulse-response func-
tion or one frequency response function at a time. In this case, only the temporal
characteristic is used, and, as might be expected, only temporal characteristics (modal
frequencies) can be estimated from the single measurement. The global characteris-
tic of modal frequency cannot be enforced. In practice, when multiple measurements
are taken, the modal frequency does not change from one measurement to the next.
Other modal parameter estimation algorithms utilize the data in a plane of the
characteristic space. For example, this corresponds to the data taken at a number of
response points but from a single excitation point or reference. This representation
of a column of measurements is shown in Fig. 21.16 as a plane in the characteristic
space. For this case, representing a single input (reference), while it is now possible
to enforce the global modal frequency assumption, it is not possible to compute
repeated roots and it is difficult to separate closely coupled modes because of the
lack of spatial data.
Many modal identification algorithms utilize data taken at a large number of out-
put DOFs due to excitation at a small number of input DOFs. Data taken in this
manner are consistent with a multiexciter type of test. Conceptually, this is repre-
sented by several planes of data parallel to the plane of data represented in Fig.
21.16. Some modal identification algorithms utilize data taken at a large number of
input DOFs and a small number of output DOFs. Data taken in this manner are con-
sistent with a roving hammer type of excitation with several fixed output sensors.
These data can also be generated by transposing the data matrix acquired using a
multiexciter test. The conceptual representation is several rows of the potential
measurement matrix perpendicular to the plane of data represented in Fig. 21.16.
Measurement data spaces involving many planes of measured data are the best pos-
sible modal identification situations, since the data subspace includes contributions
from temporal and spatial characteristics. This allows the best possibility of estimat-
ing all the important modal parameters. The data which define the subspace need to
be acquired through a consistent measurement process in order for the algorithms
to estimate accurate modal parameters. This means that the data must be measured
simultaneously and requires that data acquisition, digital signal processing, and
instrumentation be designed and operate accordingly.
EXPERIMENTAL MODAL ANALYSIS 21.45
8434_Harris_21_b.qxd 09/20/2001 12:09 PM Page 21.45