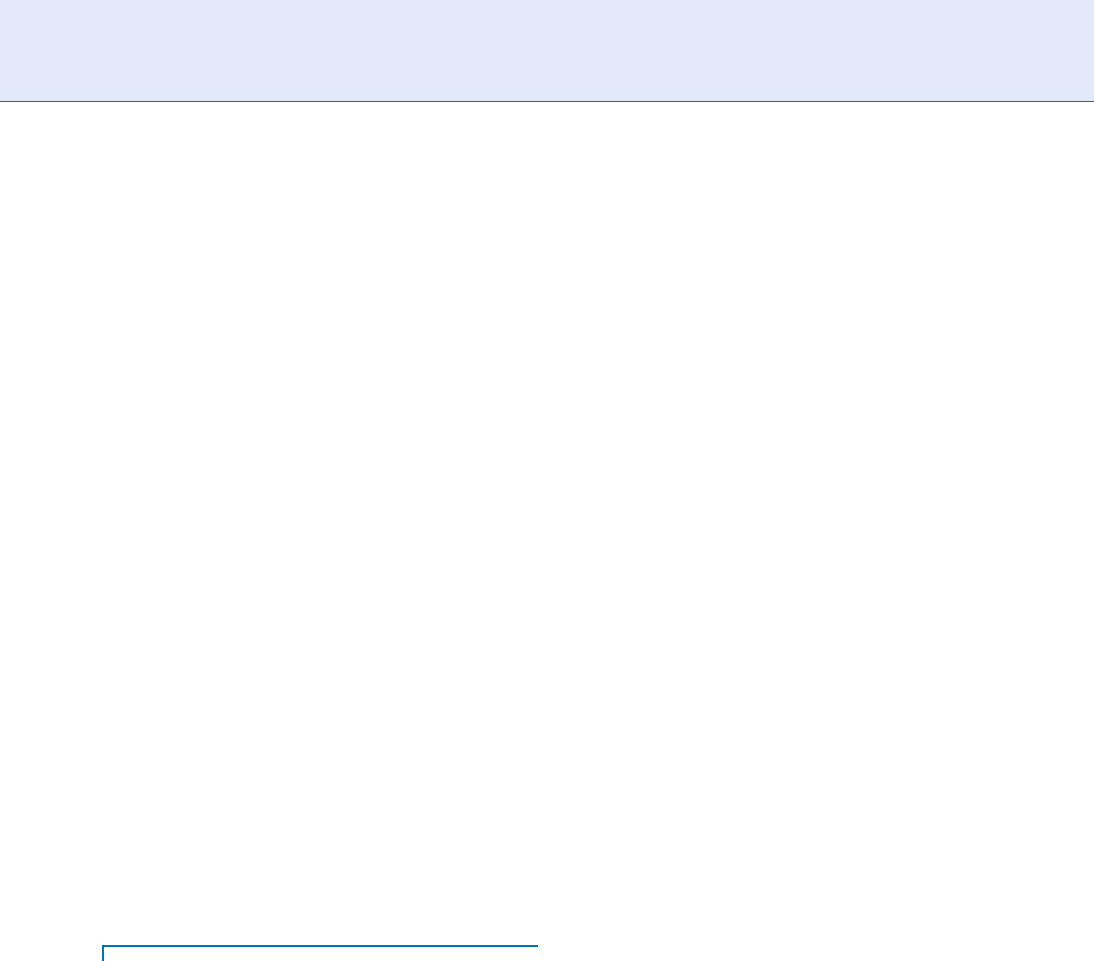
6. Snelick, R., Uludag, U., Mink, A., Indovina, M., Jain, A.K.: Large
Scale evaluation of multimodal biometric authentication using
state-of-the-art systems. IEEE Trans. Pattern Analy. Mach. Intell.
27, 450–455 (2005)
7. Hampel, F.R., Rousseeuw, P.J., Ronchetti, E.M., Stahel, W.A.:
Robust Statistics: The Approach Based on Influence Functions.
Wiley, New York (1986)
8. Mosteller, F., Tukey, J.W.: Data Analysis and Regression: A Sec-
ond Course in Statistics. Addison-Wesley, Reading, MA, USA
(1977)
9. Brunelli, R., Falavigna, D.: Person Identification using multiple
cues. IEEE Trans. Pattern Analy. Mach. Intell. 17, 955–966 (1995)
10. Verlinde, P., Cholet, G.: Comparing decision fusion paradigms
using k-NN based classifiers, decision trees and logistic regres-
sion in a multi-modal identity verification application. In: Pro-
ceedings of Second International Conference on Audio- and
Video-Based Biometric Person Authentication (AVBPA), pp.
188–193. Washington D.C., USA (1999)
11. Chatzis, V., Bors, A.G., Pitas, I.: Multimodal decision-level fu-
sion for person authentication. IEEE Trans. Syst. Man Cybernet.
Part A: Syst. Humans 29, 674–681 (1999)
12. Ben-Yacoub, S., Abdeljaoued, Y., Mayoraz, E.: Fusion of face and
speech data for person identity verification. IEEE Trans. Neural
Networks 10, 1065–1075 (1999)
13. Bigun, E.S., Bigun, J., Duc, B., Fischer, S.: Expert Conciliation for
multimodal person authentication systems using bayesian sta-
tistics. In: First International Conference on Audio- and Video-
based Biometric Person Authentication (AVBPA), pp. 291–300.
Crans-Montana, Switzerland (1997)
14. Sanderson, C., Paliwal, K.K.: Information Fusion and Person
Verification Using Speech and Face Information. Research
Paper IDIAP-RR 02-33, IDIAP (2002)
Fusion, Sensor-Level
AFZEL NOORE,RICHA SINGH,MAYANK VASTA
West Virginia University, Morgantown, WV, USA
Synonyms
Fusion, data level; Fusion, image level
Definition
Sensor level fusion combines raw biometric informa-
tion that can account for inter-class and intra-class
variability and facilitate decision making based on the
fused raw information. A typical sensor level fusion
algorithm first integrates raw biometric data either
obtained from different viewpoints (for example,
mosaicing several fingerprint impressions) or obtained
from different sensors (for example, multimodal bio-
metric images). The integrated data is then processed
and discriminatory biometric features are extracted for
matching. This level of fusion can be operated in both
verification and
▶ identification modes. Few examples
of sensor level fusion are: fingerprint mosaicing, multi-
spectral face image fusion, and multimodal biometric
image fusion.
Introduction
The concept of biometric information fusion is moti-
vated from classical multi-classifier systems that com-
bine information from different sources and represent
using a single entity. Performance driven systems that
use multiple biometric characteristics are known in
multibiometric system [1]. These systems have several
advantages over unimodal biometric systems such as
tolerance to noise and malfunction, universality, and
improved accuracy. Multibiometric systems are broadly
classified into five levels of fusion.
1. Sensor level fusion. Raw data obtained directly from
the sensors are fused without any feature extraction
and represented as a single unit. This level of fusion
is also known as data level fusion or image level
fusion (for image based biometrics).
2. Feature level fusion . Data obtained from different
sensors are first subjected to feature extraction
algorithms and the feature sets are combined to
generate a new feature vector which is subsequently
used for recognition.
3. Match score level fusion. Features extracted from
individual biometric modalities are first matched
to compute the corresponding match scores. Match
scores obtained from different biometric systems
are then combined to generate a fused match score.
4. Decision level fusion. Decisions of individual
biometric classifiers are fused to compute a com-
bined decision. This level of fusion is also known
as abstract level fusion because it is used when there
is access to only decisions from individual classifier’s.
5. Rank level fusion. With identification systems, rank
level fusion involves combining identification ranks
obtained from multiple unimodal biometrics. The
output of rank level fusion is a consolidated rank
that is used for final decision.
616
F
Fusion, Sensor-Level