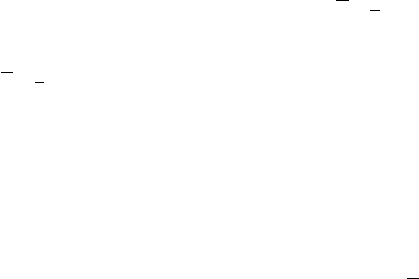
9.5 General Results for Sample Average Approximation and Sequential Sampling 411
Many other results along these lines are possible (see, e.g.,
Dupaˇcov´a and Wets [1988]). They often concern the stability of the solutions with
respect to the underlying probability distribution. For example, one might only have
observations of some random parameter but may not know the parameter’s distri-
bution. This type of analysis appears in Dupaˇcov´a [1984], R¨omisch and Schultz
[1991a], and the survey in Dupaˇcov´a [1990].
Another useful result is to have asymptotic properties of the optimal approxi-
mation value. For this, suppose that z
∗
is the optimal value of (5.1)and z
ν
is the
random optimal value of (5.2). We use properties of g and
ξ
i
so that each g(x,
ξ
i
)
is an independent and identically distributed observation of g(x, ξ) ,and g(x,ξ)
has finite variance, Var(g(x)) =
Ξ
|g(x,ξ)|
2
P(dξ) −(Eg(x,ξ))
2
. We can thus ap-
ply the central limit theorem to state that
√
ν
1
ν
∑
ν
i=1
g(x,
ξ
i
) −
Ξ
g(x,ξ)P(dξ)
converges to a random variable with distribution, N(0, Var (g(x))) . Moreover, with
the condition in Theorem 5, the random function on x defined by
√
ν
1
ν
∑
ν
i=1
g(x,
ξ
i
) −
Ξ
g(x,ξ)P(dξ)
is continuous. We can then derive the fol-
lowing result of Shapiro [1991, Theorem 3.3].
Theorem 6. Suppose that X is compact and g satisfies the following conditions:
i. g(x,·) is measurable for all x ∈ X;
ii. there exists some a :
Ξ
→ ℜ ,
Ξ
|a(ξ)|
2
P(dξ) < ∞ , |g(x
1
,
ξ
) −g(x
2
,
ξ
)|≤
a(
ξ
)|x
1
−x
2
|, for all x
1
,x
2
∈X;
iii. for some x
0
∈ X,
g(x
0
,ξ)P(dξ) < ∞ ;
and Eg(x) has a unique minimizer x
0
∈X.Then
√
ν
[z
ν
−z
∗
] converges in distri-
bution to a normal N(0, Va r g(x
0
)) .
Further results along these lines are possible using the specific structure of g
for the recourse problem as in (3.1.1). For example, if K
1
is bounded and Q has
a strong convexity property, R¨omisch and Schultz [1991b] show that the distance
between the optimizing sets in (5.1)and(5.2) can be bounded.
Given the results in Theorems 5 and 6 and some bounds on the variances and
covariances, one can construct asymptotic confidence intervals for solutions using
(5.2). We discuss this use in a sequential sampling method below. In addition, note
that all previous discrete methods can be applied to (5.2) to obtain solutions as
ν
increases. Various procedures can be used to increment
ν
and solving the resulting
approximation (5.2) using a previous solution.
Stronger results than Theorem 6 are possible when the minimum in (5.1)isa
sharp minimum in the following sense:
Eg(x, ξ) ≤ Eg(x
∗
,ξ)+kx −x
∗
, (5.7)
for some k > 0forallx ∈ X . In this case, with probability one, x
ν
= x
∗
for all
ν
sufficiently large, i.e., the convergence is exact, and, for two-stage stochastic linear
programs with relatively complete recourse, the rate of convergence is exponentially
fast, i.e., the probability of not converging in
ν
iterations is bounded by
α
e
−
βν
for
some constants
α
> 0and
β
> 0 (Shapiro and Homem-de-Mello [2000]). Similar