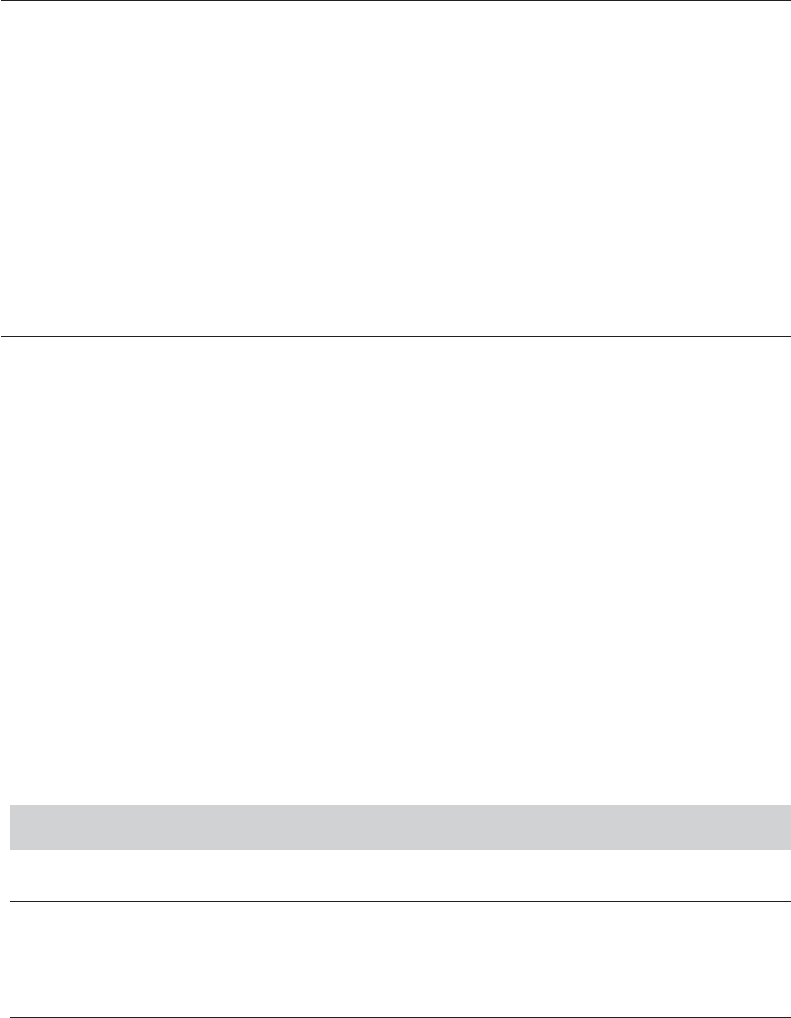
572
PART III
✦
Estimation Methodology
We used Newton’s method for the optimization, with starting values as suggested earlier.
The five iterations are as follows:
Variable Constant Age Educ Income Kids
Start values: 0.11580e+01 0.00000e+00 0.00000e+00 0.00000e+00 0.00000e+00
1st derivs. −0.25191e−08 −0.61777e+05 0.73202e+04 0.42575e+04 0.16464e+04
Parameters: 0.11580e+01 0.00000e+00 0.00000e+00 0.00000e+00 0.00000e+00
Iteration 1 F = 0.6287e+05 g
inv(H)g = 0.4367e+02
1st derivs. 0.48616e+03 −0.22449e+05 −0.57162e+04 −0.17112e+04 −0.16521e+03
Parameters: 0.11186e+01 0.17563e−01 −0.50263e−01 −0.46274e−01 −0.15609e+00
Iteration 2 F = 0.6192e+05 g
inv(H)g = 0.3547e+01
1st derivs. −0.31284e+01 −0.15595e+03 −0.37197e+02 −0.10630e+02 −0.77186e+00
Parameters: 0.10922e+01 0.17981e−01 −0.47303e−01 −0.46739e−01 −0.15683e+00
Iteration 3 F= 0.6192e+05 g
inv(H)g = 0.2598e−01
1st derivs. −0.18417e−03 −0.99368e−02 −0.21992e−02 −0.59354e−03 −0.25994e−04
Parameters: 0.10918e+01 0.17988e−01 −0.47274e−01 −0.46751e−01 −0.15686e+00
Iteration 4 F= 0.6192e+05 g
inv(H)g = 0.1831e−05
1st derivs. −0.35727e−11 0.86745e−10 −0.26302e−10 −0.61006e−11 −0.15620e−11
Parameters: 0.10918e+01 0.17988e−01 −0.47274e−01 −0.46751e−01 −0.15686e+00
Iteration 5 F= 0.6192e+05 g
inv(H)g = 0.1772e−12
Convergence based on the LM criterion, g
H
−1
g is achieved after the fourth iteration. Note
that the derivatives at this point are extremely small, albeit not absolutely zero. Table 14.7
presents the maximum likelihood estimates of the parameters. Several sets of standard er-
rors are presented. The three sets based on different estimators of the information matrix
are presented first. The fourth set are based on the cluster corrected covariance matrix
discussed in Section 14.8.4. Because this is actually an (unbalanced) panel data set, we
anticipate correlation across observations. Not surprisingly, the standard errors rise sub-
stantially. The partial effects listed next are computed in two ways. The “Average Partial
Effect” is computed by averaging λ
i
β across the individuals in the sample. The “Partial
Effect” is computed for the average individual by computing λ at the means of the data.
The next-to-last column contains the ordinary least squares coefficients. In this model,
there is no reason to expect ordinary least squares to provide a consistent estimator of
β. The question might arise, What does ordinary least squares estimate? The answer is the
slopes of the linear projection of DocVis on x
it
. The resemblance of the OLS coefficients
to the estimated partial effects is more than coincidental, and suggests an answer to the
question.
The analysis in the table suggests three competing approaches to modeling DocVis. The
results for the geometric regression model are given in Table 14.7. At the beginning of this
section, we noted that the more conventional approach to modeling a count variable such as
DocVis is with the Poisson regression model. The log-likelihood function and its derivatives
TABLE 14.7
Estimated Geometric Regression Model Dependent Variable: DocVis:
Mean
=
3.18352, Standard Deviation
=
5.68969
St. Er. St. Er. St. Er. St. Er. PE
Variable Estimate H E[H] BHHH Cluster APE Mean OLS Mean
Constant 1.0918 0.0524 0.0524 0.0354 0.1112 — — 2.656
Age 0.0180 0.0007 0.0007 0.0005 0.0013 0.0572 0.0547 0.061 43.52
Education −0.0473 0.0033 0.0033 0.0023 0.0069 −0.150 −0.144 −0.121 11.32
Income −0.0468 0.0041 0.0042 0.0023 0.0075 −0.149 −0.142 −0.162 3.52
Kids −0.1569 0.0156 0.0155 0.0103 0.0319 −0.499 −0.477 −0.517 0.40