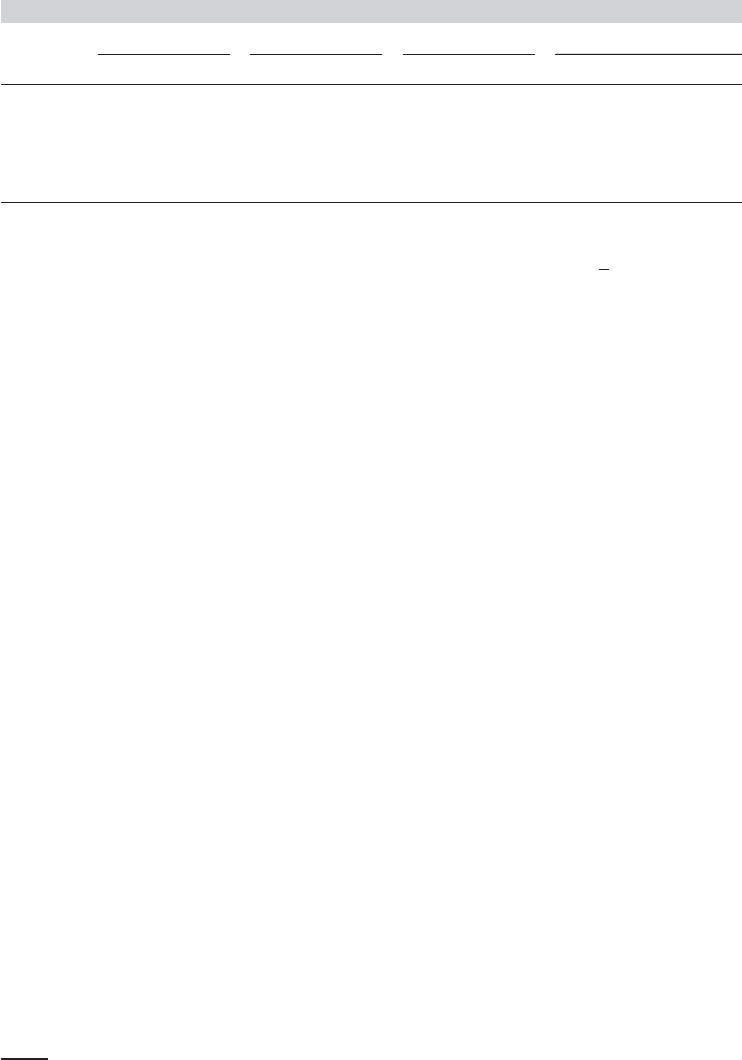
CHAPTER 17
✦
Discrete Choice
695
TABLE 17.1
Estimated Probability Models
Linear Logistic Probit Complementary log log
Variable Coef ficient Slope Coefficient Slope Coef ficient Slope Coef ficient Slope
Constant −1.498 — −13.021 — −7.452 — −10.631 —
GPA 0.464 0.464 2.826 0.534 1.626 0.533 2.293 0.477
TUCE 0.010 0.010 0.095 0.018 0.052 0.017 0.041 0.009
PSI 0.379 0.379 2.379 0.450 1.426 0.468 1.562 0.325
f (
¯
x
ˆ
β) 1.000 0.189 0.328 0.208
and logit models.
12
One indicator is a comparison of the coefficients. In view of the different
variances of the distributions, one for the normal and π
2
/3 for the logistic, we might expect to
obtain comparable estimates by multiplying the probit coefficients by π/
√
3 ≈ 1.8. Amemiya
(1981) found, through trial and error, that scaling by 1.6 instead produced better results. This
proportionality result is frequently cited. The result in (17-11) may help to explain the finding.
The index x
β is not the random variable. The marginal effect in the probit model for, say, x
k
is
φ( x
β
p
)β
pk
, whereas that for the logit is (1−)β
lk
. (The subscripts p and l are for probit and
logit.) Amemiya suggests that his approximation works best at the center of the distribution,
where F = 0.5, or x
β = 0 for either distribution. Suppose it is. Then φ(0) = 0.3989 and
(0)[1− (0)] = 0.25. If the marginal effects are to be the same, then 0.3989 β
pk
= 0.25β
lk
,
or β
lk
= 1.6β
pk
, which is the regularity observed by Amemiya. Note, though, that as we
depart from the center of the distribution, the relationship will move away from 1.6. Because
the logistic density descends more slowly than the normal, for unbalanced samples such as
ours, the ratio of the logit coefficients to the probit coefficients will tend to be larger than 1.6.
The ratios for the ones in Table 17.1 are closer to 1.7 than 1.6.
The computation of the derivatives of the conditional mean function is useful when the vari-
able in question is continuous and often produces a reasonable approximation for a dummy
variable. Another way to analyze the effect of a dummy variable on the whole distribution is
to compute Prob(Y = 1) over the range of x
β (using the sample estimates) and with the two
values of the binary variable. Using the coefficients from the probit model in Table 17.1, we
have the following probabilities as a function of GPA, at the mean of TUCE:
PSI = 0: Prob( GRADE = 1) = [−7.452 + 1.626GPA + 0.052( 21.938)],
PSI = 1: Prob( GRADE = 1) = [−7.452 + 1.626GPA + 0.052( 21.938) + 1.426].
Figure 17.2 shows these two functions plotted over the range of GPA observed in the sample,
2.0 to 4.0. The marginal effect of PSI is the difference between the two functions, which ranges
from only about 0.06 at GPA = 2 to about 0.50 at GPA of 3.5. This effect shows that the
probability that a student’s grade will increase after exposure to PSI is far greater for students
with high GPAs than for those with low GPAs. At the sample mean of GPA of 3.117, the effect
of PSI on the probability is 0.465. The simple derivative calculation of (17-12) is given in Table
17.1; the estimate is 0.468. But, of course, this calculation does not show the wide range of
differences displayed in Figure 17.2.
Table 17.2 presents the estimated coefficients and marginal effects for the probit and
logit models in Table 17.2. In both cases, the asymptotic covariance matrix is computed
from the negative inverse of the actual Hessian of the log-likelihood. The standard errors for
the estimated marginal effect of PSI are computed using (17-24) and (17-25) since PSI is a
binary variable. In comparison, the simple derivatives produce estimates and standard errors
of (0.449, 0.181) for the logit model and (0.464, 0.188) for the probit model. These differ only
slightly from the results given in the table.
12
One might be tempted in this case to suggest an asymmetric distribution for the model, such as the Gumbel
distribution. However, the asymmetry in the model, to the extent that it is present at all, refers to the values
of ε, not to the observed sample of values of the dependent variable.