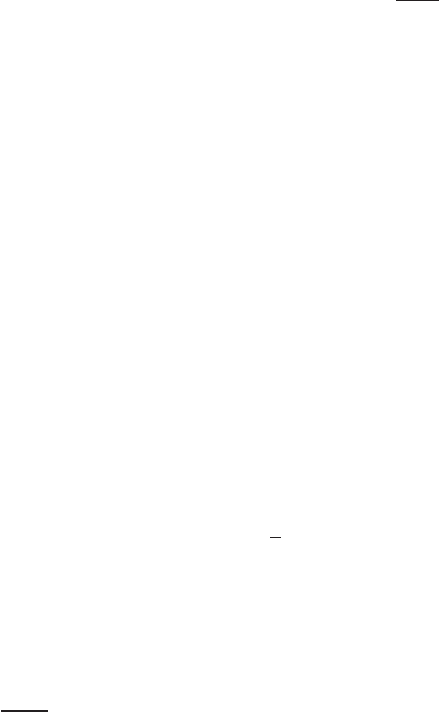
CHAPTER 17
✦
Discrete Choice
701
Estimation of the model produces an estimate of β
7
of −0.00112. The naive average partial
effect for x
7
is −0.000254. This is the first part in the earlier decomposition. The second,
functional form term (averaged over the sample observations) is 0.0000634, so the estimated
interaction effect, the sum of the two terms is −0.000191. The naive calculation errs by about
(−0.000254/ − 0.000191 − 1) × 100 percent = 33 percent.
17.3.3 MEASURING GOODNESS OF FIT
There have been many fit measures suggested for QR models.
14
At a minimum, one
should report the maximized value of the log-likelihood function, ln L. Because the hy-
pothesis that all the slopes in the model are zero is often interesting, the log-likelihood
computed with only a constant term, ln L
0
[see (17-29)], should also be reported. An ana-
log to the R
2
in a conventional regression is McFadden’s (1974) likelihood ratio index,
LRI = 1 −
ln L
ln L
0
.
This measure has an intuitive appeal in that it is bounded by zero and one. (See Sec-
tion 14.6.5.) If all the slope coefficients are zero, then it equals zero. There is no way
to make LRI equal 1, although one can come close. If F
i
is always one when y equals
one and zero when y equals zero, then ln L equals zero (the log of one) and LRI equals
one. It has been suggested that this finding is indicative of a “perfect fit” and that LRI
increases as the fit of the model improves. To a degree, this point is true. Unfortunately,
the values between zero and one have no natural interpretation. If F(x
i
β) is a proper
cdf, then even with many regressors the model cannot fit perfectly unless x
i
β goes to
+∞ or −∞. As a practical matter, it does happen. But when it does, it indicates a flaw
in the model, not a good fit. If the range of one of the independent variables contains
a value, say, x
∗
, such that the sign of (x − x
∗
) predicts y perfectly and vice versa, then
the model will become a perfect predictor. This result also holds in general if the sign
of x
β gives a perfect predictor for some vector β.
15
For example, one might mistakenly
include as a regressor a dummy variable that is identical, or nearly so, to the dependent
variable. In this case, the maximization procedure will break down precisely because
x
β is diverging during the iterations. [See McKenzie (1998) for an application and
discussion.] Of course, this situation is not at all what we had in mind for a good fit.
Other fit measures have been suggested. Ben-Akiva and Lerman (1985) and Kay
and Little (1986) suggested a fit measure that is keyed to the prediction rule,
R
2
BL
=
1
n
n
i=1
y
i
ˆ
F
i
+ (1 − y
i
)(1 −
ˆ
F
i
)
,
which is the average probability of correct prediction by the prediction rule. The diffi-
culty in this computation is that in unbalanced samples, the less frequent outcome will
usually be predicted very badly by the standard procedure, and this measure does not
pick up that point. Cramer (1999) has suggested an alternative measure that directly
14
See, for example, Cragg and Uhler (1970), Amemiya (1981), Maddala (1983), McFadden (1974), Ben-Akiva
and Lerman (1985), Kay and Little (1986), Veall and Zimmermann (1992), Zavoina and McKelvey (1975),
Efron (1978), and Cramer (1999). A survey of techniques appears in Windmeijer (1995).
15
See McFadden (1984) and Amemiya (1985). If this condition holds, then gradient methods will find that β.