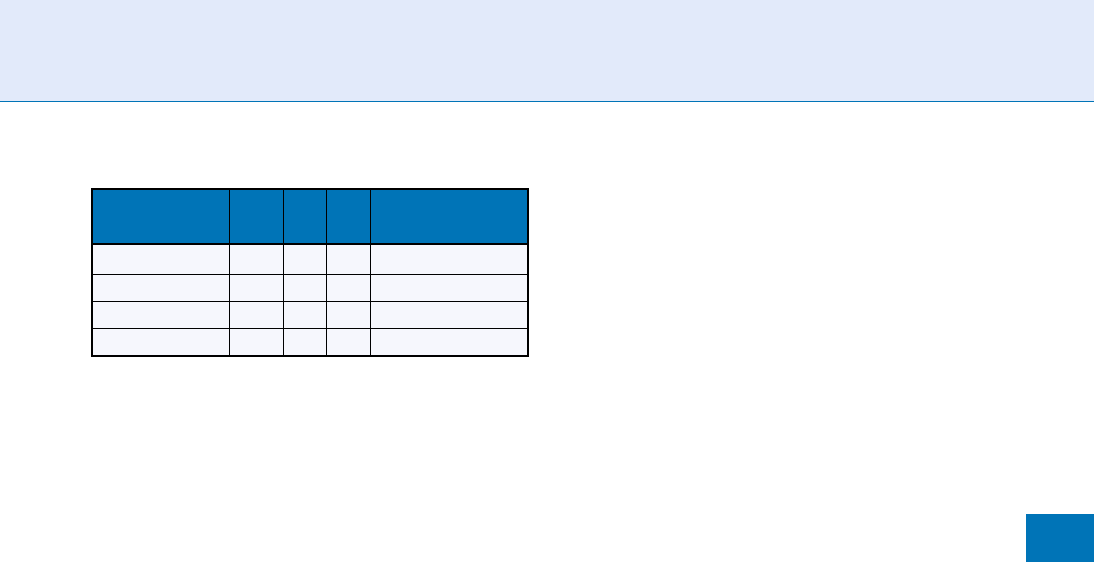
However, the improvement is very slight in some
cases, e.g., for the Yale database.
2. The BHE or the introduced algorithm can improve
the recognition rates significantly. The BHE leads
to an improvement varying from 29.9 to 45.4%,
and in the case of the introduced algorithm it varies
from 53.3 to 62.6%. In other words, these two
methods are both useful in eliminating the effect
of uneven illumination on face recognition. In
addition, the introduced algorithm can achieve
the best performance level of all the methods used
in the experimen t.
3. The BHE method is very simple and does not
require any prior knowledge. Compared to the
traditional local contrast enhancement methods
[5], its computational burden is for less. The
main reason for this is that all the pixels within a
block are equalized in the process, rather than just a
single pixel, as in the adaptive block enhancement
method. Nevertheless, as in the case of the tradi-
tional local contrast enhancement methods, noise
is amplified after this process.
4. If the images processed by the HE are adopted
to estimate the illumination category, the cor-
responding recognition rates using the different
databases will be lowered. This is because variations
between the images are affected not only by the
illumination, but also by other facto rs, such as
age, gender, race, and makeup. The illumination
map can eliminate the distinctive personal infor-
mation to the extent possible, while keeping the
illumination information unchanged. Therefore,
the illumination category can be estimated more
accurately, and a more suitable illumination mode
is selected.
The reconstructed facial images using the intro-
duced algorithm appear to be very natural, and display
great visual improvement and lighting smoothness.
The effect of uneven lighting, including shadows, is
almost eliminated. However, if there are glasses or a
mustache, which are not Lambertian surface, in an
image, some side effects may be seen under some
special light source models. For instance, the glasses
may disappear or the mustache may become faint.
Summary
This essay discussed a model-based method, which can
be used for illumination compensation in face recog-
nition. For a query image, the illumination category is
first evaluated, followed by shape normalization, then
the corresponding lighting model is used to compen-
sate for uneven illumination. Next, the reconstructed
texture is mapped backwards from the reference
shape to that of the original shape in order to build
an image under normal illumination. This lighting com-
pensation approach is not only useful for face recogni-
tion when the faces are under varying illumination,
but can also be used for face reconstruction. More im-
portantly, the images of a query input are not required
for training. In the introduced algorithm, 2D face shape
model is adopted in order to address the effect of differ-
ent geometries or shapes of human faces. Therefore, a
more reliable and exact reconstruction of the human
face is possible, and the reconstructed face is under
normal illumination and appears more natural visually.
Experimental results revealed that preprocessing the
faces using the lighting compensation algorithm greatly
improves the recognition rate.
Related Entries
▶ Face Recognition, Over view
References
1. Adini, Y., Moses, Y., Ullman, S.: Face recognition: the problem
of compensating for changes in illumination direction. IEEE T.
Pattern Anal. 19(7), 721–732 (1997)
2. Turk, M., Pentland, A.: Eigenfaces for recognition. J. Cognitive
Neurosci. 3, 71–86 (1991)
3. Bartlett, M.S., Movellan, J.R., Sejnowski, T.J.: Face recognition by
independent component analysis. IEEE T. Neural Networ. 13(6),
1450–1464 (2002)
4. Yale University [Online]. Available at: http://cvc.yale.edu/
projects/yalefacesB/yalefacesB.html
5. Pizer, S.M., Amburn, E.P.: Adaptive histogram equalization and
its variations. Comput. Vision Graph. 39, 355–368 (1987)
Illumination Compensation. Table 1 Face recognition
results using deferent preprocessing methods
Recognition
rate (%) None HE BHE
The introduced
method
YaleB 43.4 61.4 77.5 99.5
Yale 36.7 36.7 80.0 90.0
AR 25.9 37.7 71.3 81.8
Combined 30.1 32.2 60.0 92.7
Illumination Compensation
I
721
I