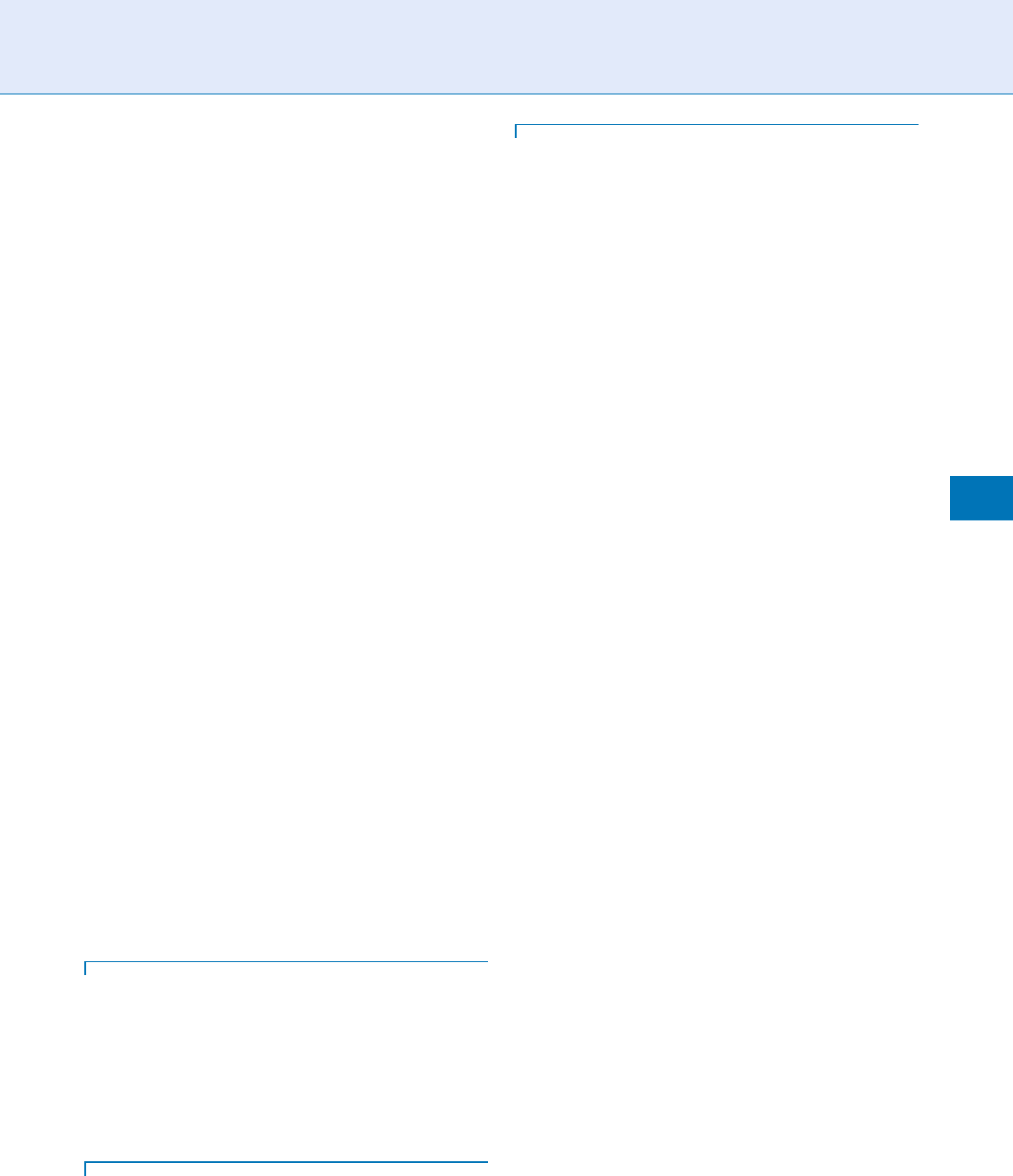
4. Vijaya Kumar, B.V.K., Savvides, M., Venkataramani, K., Xie, C.:
Spatial frequency domain image processing for biometric recog-
nition. In: Proceedings of International Conference on Image
Processing, September 2002, pp. 53–56
5. Savvides, M., Vijaya Kumar, B.V.K.: Efficient design of advanced
correlation filters for robust distortion-tolerant face recognition.
In: Proceedings of IEEE Conference on Advanced Video and
Signal Based Surveillance, July 2003, pp. 45–52
6. Thornton, J., Savvides, M., Vijaya Kumar, B.V.K.: Robust iris
recognition using advanced correlation techniques. In: Proceed-
ings of International Conference on Image Analysis and Recog-
nition, September 2005, pp. 1098–1105
7. Vijaya Kumar, B.V.K., Mahalanobis A., Juday, R.D.: Correlation
Pattern Recognition. Cambridge University Press, Cambridge,
UK (2005)
8. Vijaya Kumar, B.V.K.: Tutorial survey of composite filter designs
for optical correlators. Appl. Opt. 31, 4773–4801 (1992)
9. Vijaya Kumar, B.V.K., Mahalanobis, A., Takessian, A.: Optimal
tradeoff circular harmonic function corr1elation filter methods
providing controlled in-plane rotation response. IEEE Trans.
Image Process. 9(6), 1025–1034 (2000)
10. Kerekes, R., Vijaya Kumar, B.V.K.: Correlation filters with con-
trolled scale response. IEEE Trans. Image Process. 15(7),
1794–1802 (2006)
11. Mahalanobis, A., Vijaya Kumar, B.V.K., Casasent, D.: Minimum
average correlation energy filters. Appl. Opt. 26, 3630–3633
(1987)
12. Vijaya Kumar, B.V.K., Carlson, D.W., Mahalanobis, A.: Optimal
trade-off synthetic discriminant function filters for arbitrary
devices. Opt. Lett. 19, 1556–1558 (1994)
13. Perez, P.: Markov random fields and images. CWI Quarterly
11(4), 413–437 (1998)
14. Felzenszwalb, P., Huttenlocher, D.: Markov random fields and
images. Int. J. Comput. Vis. 70(1), 41–54 (2006)
15. ICE iris database. National Institute of Standards and Technolo-
gy. http://iris.nist.gov/ICE/, 2005. Accessed 1 March 27, 2009
16. Daugman, J.: How Iris Recognition Works. IEEE Trans. Circ.
Syst. video Tech. 14(1), 21–30 (2004)
Iris Recognition with Deformation
and Occlusion Estimation
▶ Iris Recognition Using Correlation Filters
Iris Retina Biometric Fusion
▶ Simultaneous Capture of Iris and Retina for
Recognition
Iris Sample Synthesis
NATALIA A. S CHMID
Department of Computer Science and Electrical
Engineering, West Virginia University, Morgantown,
WV, USA
Synonym
Synthetic iris images
Definition
Iris image synthesis is a process of creating images of
an iris by means of statistical and stochastic models,
computer graphic tool s or through manipulating,
modifying or transforming parts or complete images
collected from real irises. Since the size of synthesized
datasets can be made arbitrary large, these data are
suggested to be used for the purpose of extensive
testing of the performance and efficiency of newly
designed iris recognition algorithms.
Introduction
Iris as a biometric has been known for a long time.
However, only in the recent years it has gained a sub-
stantial attention of both the research community and
governmental organizations resulting in the develop-
ment of a large number of new iris encoding and proces-
sing algorithms. Most of the designed systems and
algorithms are claimed to have exclusively high recogni-
tion performance. However, since there are no publicly
available large-scale and even medium-size datasets, only
very few newly designed algorithms have undergone
extensive testing. There are several datasets of frontal
view iris images presently available for public use. A
brief summary of these databases is provided in Table 1.
With the lack of data, two major solutions to the
problem of algorithm testing are possible: (1) physically
collecting a large number of iris images or (2) syntheti-
cally generating a
▶ large scale dataset of iris images.
This article addresses the second approach. The
following sections summarize a number of techniques
to synthesize iris images and characterize methods to
evaluate the performance of generated images.
Iris Sample Synthesis
I
843
I