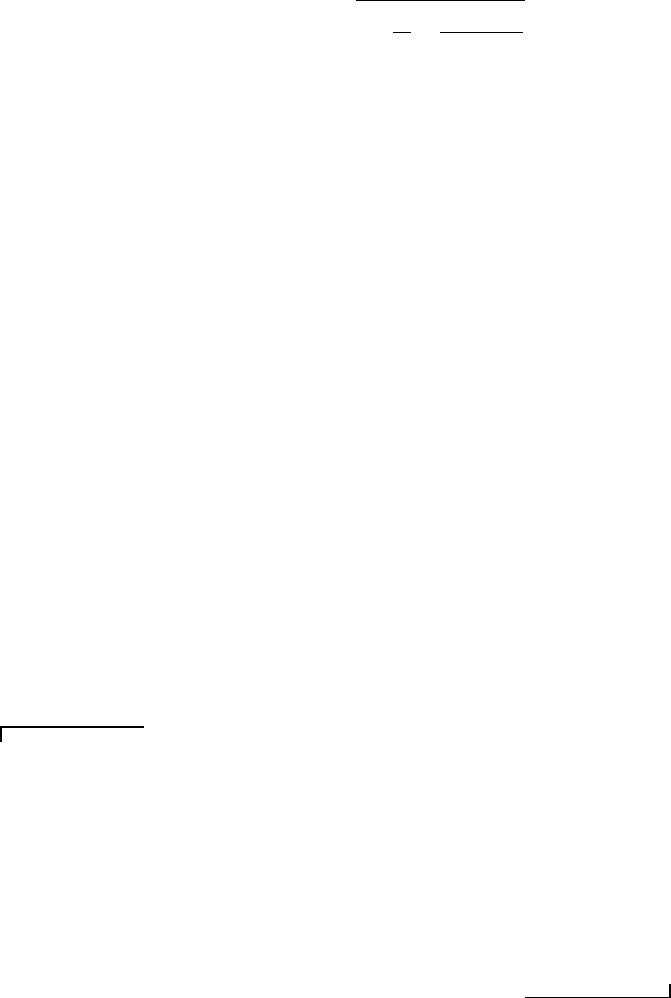
Regression and Correlation 307
y
n
= y
n
c
i
± t
N−2,P
S
yx
s
1 +
1
N
+
(x
n
− ¯x)
2
S
xx
. (8.29)
This interval is marked by the hyperbolic curves labeled d (based upon
95 % confidence). Note that the hyperbolic curves are farther from the
regression line for this case than for the mean response case. This is because
Equation 8.29 estimates a single new value of y, whereas Equation 8.28
estimates the mean of a large number of y values.
Finally, the range within which a y
i
value probably will be, with respect
to its corresponding y
c
i
value, is
y
i
= y
c
i
± t
ν,P
S
yx
, (8.30)
where t
ν,P
S
yx
denotes the precision interval. This expression establishes
the confidence intervals that always should be plotted whenever a regression
line is present. Basically, Equation 8.30 defines the limits within which P
percent of a large number of measured y
i
values will be with respect to
the y
c
i
value for a given value of x
i
. Its confidence intervals are denoted
by the lines labeled c (based upon 95 % confidence) which are parallel to
the regression line. Equation 8.30 also can be used for a higher mth-order
regression fit to establish the confidence intervals. This is provided that ν
is determined by ν = N − (m + 1) and that the general expression for S
yx
given in Equation 8.25 is used.
Several useful inferences can be drawn from Equation 8.30. For a fixed
number of measurements, N, the extent of the precision interval increases as
the percent confidence is increased. The extent of the precision interval must
be greater if more confidence is required in the estimate of y
i
. For a given
confidence, as N is increased the extent of the precision interval decreases.
A smaller precision interval is required to estimate y
i
if a greater number of
measurements is acquired.
Example Problem 8.4
Statement: For the set of [x,y] data pairs [0.00, 1.15; 1.00, 3.76; 2.00, 0.41; 3.00,
1.30; 4.00, 6.42; 5.00, 6.42; 6.00, 5.20; 7.00, 7.87] determine the linear best-fit relation
using least-squares regression analysis. Then estimate at 95 % confidence the intervals
of ¯y
i
, y
n
, and y
i
according to Equations 8.28 through 8.30 for x = 2.00.
Solution: From Equation 8.28 it follows directly that ¯y
i
= 2.68 ± 1.93. That is,
there is a 95 % chance that the mean value of a large number of measured y
i
values
for x = 2.00 will be within ±1.93 of the y
c
i
value of 2.68. Further, from Equation 8.29,
y
n
= 2.68 ± 4.95, which implies that there is a 95 % chance that a new measurement
of y for x = 2.00 will be within ±4.95 of 2.68. Finally, from Equation 8.30, y
i
= 2.68
± 4.56. The confidence intervals for this data set for the range 0 ≤ x ≤ 7 are shown in
Figure 8.4.
Another confidence interval related to the regression fit can be estab-
lished for the estimate of a value of x for a given value of y. This situation