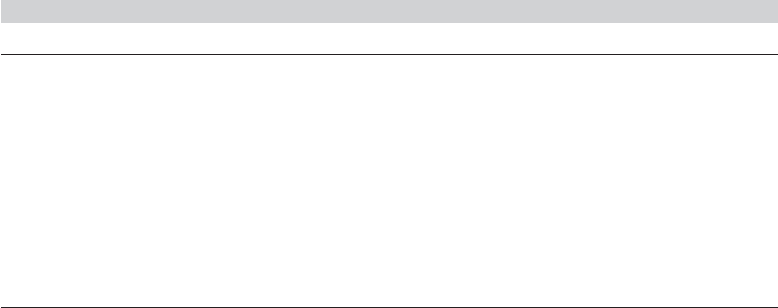
CHAPTER 17
✦
Discrete Choice
749
TABLE 17.18
Partial Effects in Gender Economics Model
Direct Indirect Total (Std. Error) (Type of Variable, Mean)
Gender Economics Equation
AcRep −0.002022 −0.001453 −0.003476 (0.001126) (Continuous, 119.242)
PctWecon +0.4491 +0.4491 (0.1568) (Continuous, 0.24787)
EconFac +0.01190 +0.1190 (0.01292) (Continuous, 6.74242)
Relig −0.06327 −0.02306 −0.08632 (0.08220) (Binary, 0.57576)
WomStud +0.1863 +0.1863 (0.0868) (Endogenous, 0.43939)
PctWfac +0.14434 +0.14434 (0.09051) (Continuous, 0.35772)
Women’s Studies Equation
AcRep −0.00780 −0.00780 (0.001654) (Continuous, 119.242)
PctWfac +0.77489 +0.77489 (0.3591) (Continuous, 0.35772)
Relig −0.17777 −0.17777 (0.11946) (Binary, 0.57576)
In all cases, standard errors for the estimated partial effects can be computed using the delta
method or the method of Krinsky and Robb.
Table 17.18 presents the estimates of the partial effects and some descriptive statistics for
the data. The calculations were simplified slightly by using the restricted model with ρ = 0.
Computations of the marginal effects still require the preceding decomposition, but they
are simplified by the result that if ρ equals zero, then the bivariate probabilities factor into
the products of the marginals. Numerically, the strongest effect appears to be exerted by
the representation of women on the faculty; its coefficient of +0.4491 is by far the largest.
This variable, however, cannot change by a full unit because it is a proportion. An increase
of 1 percent in the presence of women on the economics faculty raises the probability by
only +0.004, which is comparable in scale to the effect of academic reputation. The effect of
women on the faculty is likewise fairly small, only 0.0014 per 1 percent change. As might have
been expected, the single most important influence is the presence of a women’s studies
program, which increases the likelihood of a gender economics course by a full 0.1863. Of
course, the raw data would have anticipated this result; of the 31 schools that offer a gender
economics course, 29 also have a women’s studies program and only two do not. Note finally
that the effect of religious affiliation (whatever it is) is mostly direct.
17.5.6 ENDOGENOUS SAMPLING IN A BINARY CHOICE MODEL
We have encountered several instances of nonrandom sampling in the binary choice
setting. In Section 17.3.6, we examined an application in credit scoring in which the
balance in the sample of responses of the outcome variable, C = 1 for acceptance of
an application and C = 0 for rejection, is different from the known proportions in the
population. The sample was specifically skewed in favor of observations with C = 1
to enrich the data set. A second type of nonrandom sampling arose in the analysis
of nonresponse/attrition in the GSOEP in Example 17.17. The data suggest that the
observed sample is not random with respect to individuals’ presence in the sample
at different waves of the panel. The first of these represents selection specifically on
an observable outcome—the observed dependent variable. We constructed a model
for the second of these that relied on an assumption of selection on a set of certain
observables—the variables that entered the probability weights. We will now examine
a third form of nonrandom sample selection, based crucially on the unobservables in
the two equations of a bivariate probit model.