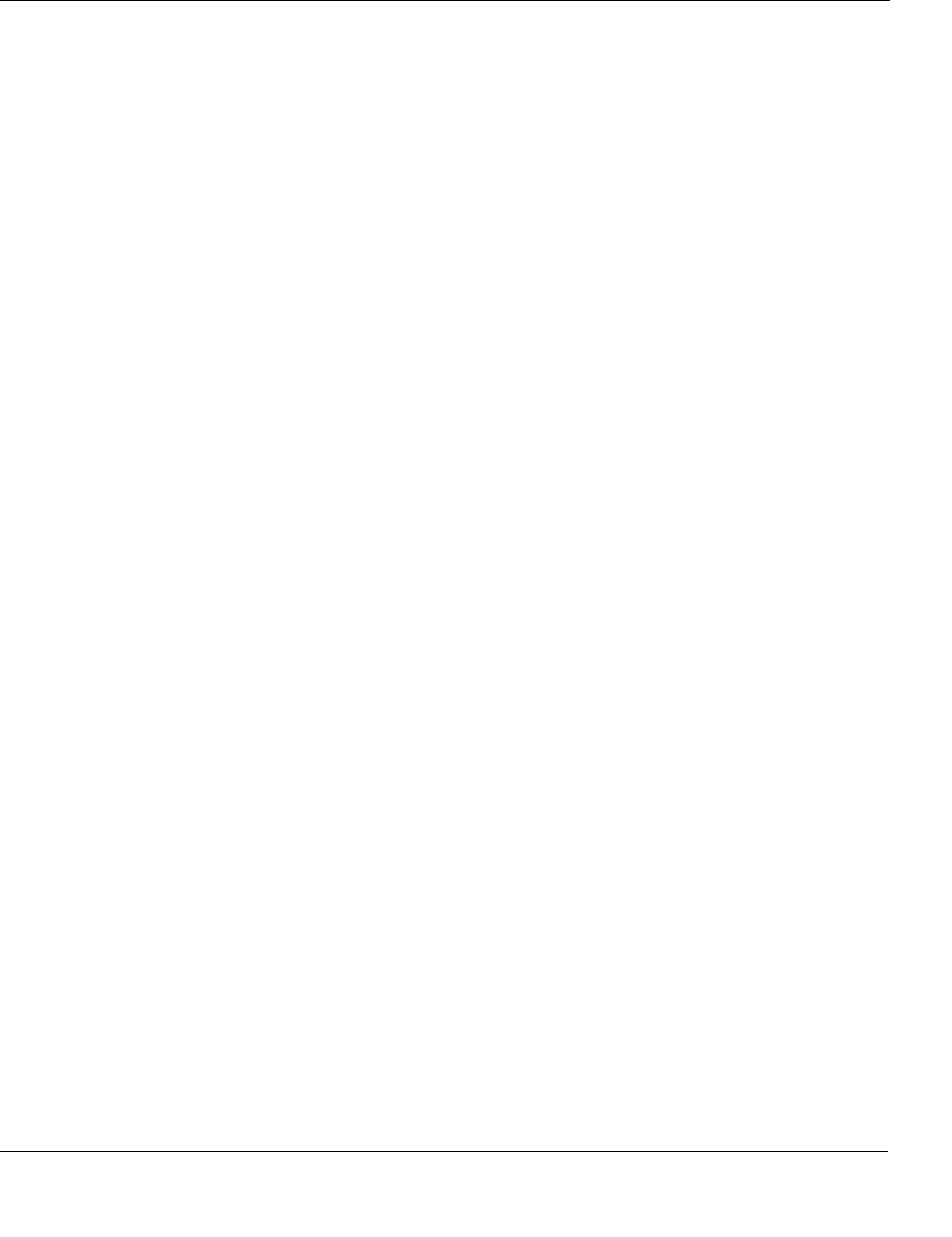
ptg6843605
facility location − factorial
Page 131 The Encyclopedia of Operations Management
Fixed-position layout (project-layout) – A fixed-position layout is used in projects where the workers,
equipment, and materials go to the production site because the product is too large, fragile, or heavy to move.
This type of layout is also called a project layout because the work is usually organized around projects. The
equipment is often left on-site because it is too expensive to move frequently. Due to the nature of the work, the
workers in a fixed position layout are usually highly skilled. The most familiar examples of a fixed position
layout are the construction of building or house. However, a fixed position layout is also used in shipbuilding
and machine repair. The major concerns with a fixed-position layout are meeting the project requirements,
within budget, and within schedule. See the project management entry.
The Theory of Constraints literature suggests that the focus for all layouts should be on the bottleneck
process. See the Theory of Constraints (TOC) entry.
See 5S, assembly line, cellular manufacturing, continuous flow, CRAFT, cross-training, experience
engineering, flowshop, focused factory, job order costing, job shop, lean thinking, line balancing, mixed model
assembly, planogram, plant-within-a-plant, process design, process map, product-process matrix, project
management, spaghetti chart, Theory of Constraints (TOC), workcenter.
facility location – The physical site for a building.
The facility location problem is to find the best locations for the organization’s facilities (warehouses, stores,
factories, offices). The facility location problem is often defined in terms of minimizing the sum of the incoming
and outgoing transportation costs. In a retail context, the problem is often defined in terms of maximizing
revenue. In the service context, the problem is defined in terms of meeting some service criterion, such as
customer travel time or response time for customer needs.
Facility location theory suggests that the problem can be broken into finite and infinite set location models.
The finite set location models evaluate a limited number of locations and determine which one is best. The
infinite set location models find the best x-y coordinates (or latitudes and longitudes) for a site (or sites) that
minimize some mathematical objective function. The center-of-gravity and numeric-analytic location models are
infinite set location models. The gravity model for competitive retail store location and the Kepner-Tregoe
Model are finite set location models.
Some location models assume that vehicles can travel directly across any geography, while others assume
that vehicles are constrained to existing transportation networks. Some models assume that cost is proportional
to the distance or time traveled, whereas others include all relevant costs, including tariffs, duties, and tolls.
See center-of-gravity model for facility location, gravity model for competitive retail store location, great
circle distance, greenfield, Kepner-Tregoe Model, numeric-analytic location model, process design, supply chain
management, tariff, warehouse.
factor analysis – A multivariate statistical method used to reduce the number of variables in a dataset without
losing much information about the correlation structure between the variables.
Factor analysis originated in psychometrics and is used in behavioral sciences, social sciences, marketing,
operations management, and other applied sciences that deal with large datasets. Factor analysis describes the
variability of a number of observed variables in terms of a fewer number of unobserved variables called factors,
where the observed variables are linear combinations of the factors plus error terms. To use an extreme example,
a study measures people’s height in both inches and centimeters. These two variables have a correlation of 100%
and the analysis can be simplified by combining the variables into one “factor” without losing any information.
Factor analysis is related to Principal Component Analysis (PCA). Because PCA performs a variance-
maximizing rotation of the variable space, it takes into account all variability in the variables. In contrast, factor
analysis estimates how much of the variability is due to common factors. The two methods become essentially
equivalent if the error terms in the factor analysis model can be assumed to all have the same variance.
Cluster analysis and factor analysis are both data reduction methods. Given a dataset with rows that are
cases (e.g., respondents to a survey) and columns that are variables (e.g., questions on a survey), cluster analysis
groups cases into clusters and factor analysis groups variables into factors. Using the survey example, cluster
analysis groups similar respondents and factor analysis groups similar variables.
See cluster analysis, Principal Component Analysis (PCA).
factorial – A mathematical function denoted as n! and defined as the product of all positive integers less than or
equal to n; in other words, n! = n · (n – 1) · (n – 2) · · · 2 · 1.