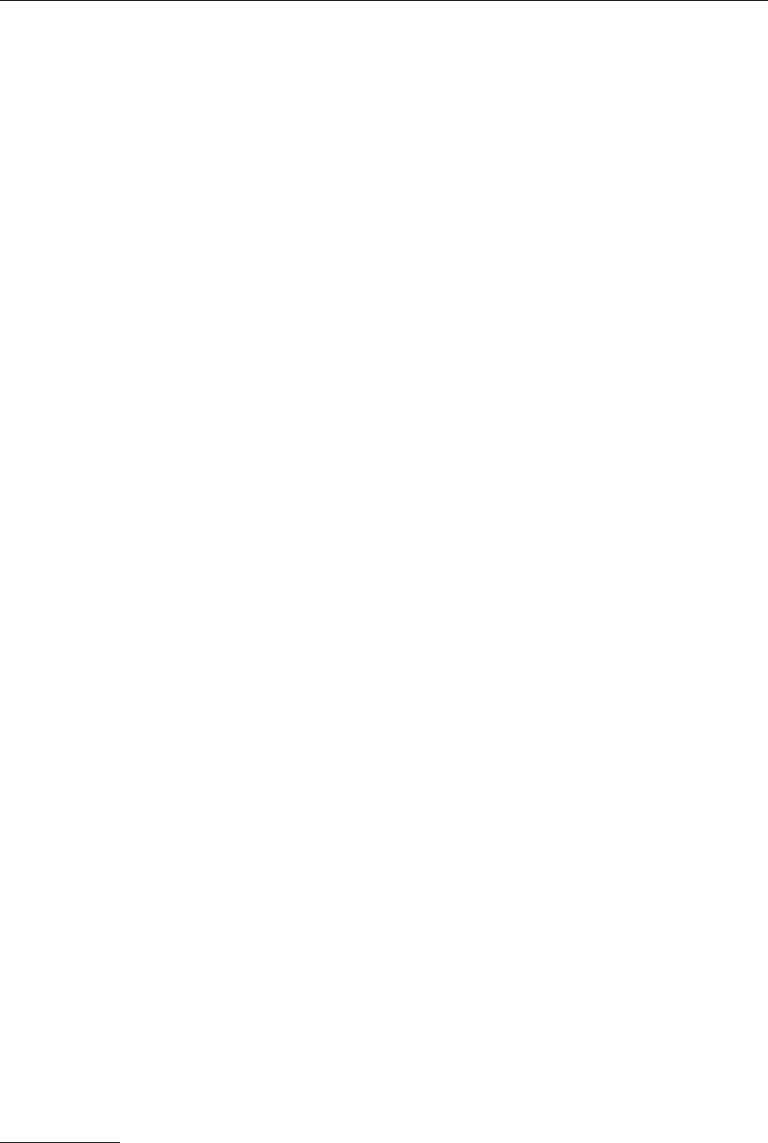
Introduction 9
tinuous. There are other statistical techniques for analyzing models with categorical
latent variables, but SEM deals with continuous latent variables only.
Latent variables in SEM generally correspond to hypothetical constructs or fac-
tors, which are explanatory variables presumed to reflect a continuum that is not directly
observable. An example is the construct of intelligence. There is no single, definitive mea-
sure of intelligence. Instead, researchers use different types of observed variables, such
as tasks of verbal reasoning or memory capacity, to assess various facets of intelligence.
Latent variables in SEM can represent a wide range of phenomena. For example, con-
structs about attributes of people (e.g., intelligence, neuroticism), higher-level units of
analysis (e.g., groups, geographic regions), or measures, such as method effects (e.g.,
self-report, observational), can all be represented as latent variables in SEM.
An observed variable used as an indirect measure of a construct is referred to as an
indicator. The explicit distinction between factors and indicators in SEM allows one to
test a wide variety of hypotheses about measurement. Suppose that a researcher believes
that variables X
1
, X
2
, and X
3
tap some common domain that is distinct from the one
assessed by X
4
and X
5
. In SEM, it is relatively easy to specify a model where X
1
–X
3
are
the indicators of one factor and X
4
–X
6
are indicators of a different factor. If the fit of
the model just described to the data is poor, then this measurement hypothesis would
be rejected. The ability to analyze both observed and latent variables distinguishes
SEM from some more standard statistical techniques, such as the analysis of variance
(ANOVA) and MR, which analyze observed variables only.
Another class of variables in SEM corresponds to residual or error terms, which can
be associated with either observed variables or factors specified as outcome (dependent)
variables. In the case of indicators, a residual term represents variance unexplained
by the factor that the corresponding indicator is supposed to measure. Part of this
unexplained variance is due to random measurement error, or score unreliability.
4
The
explicit representation of measurement error is a special characteristic of SEM. This is
not to say that SEM can compensate for gross psychometric flaws—no technique can—
but this property lends a more realistic quality to an analysis. Some more standard sta-
tistical techniques make unrealistic assumptions in this area. For example, it is assumed
in MR that all predictor variables are measured without error. In diagrams of structural
equation variables, residual terms may be represented using the same symbols as for
substantive latent variables. This is because error variance must be estimated, given the
whole model and the data; thus in this sense error variance is not directly observable in
the raw data. Also, residual terms are explicitly represented in the syntax or diagrams
of some SEM computer tools as latent variables. Even if they are not, error variance is
estimated in basically all SEM analyses, and estimates about the degree of residual vari-
ance often have interpretive import.
As already mentioned, it is possible in SEM to analyze substantive latent variables
4
The other part of unexplained variance is systematic (i.e., reliable) but unrelated to the underlying
construct. Another term for this part of residual variance is specific variance.