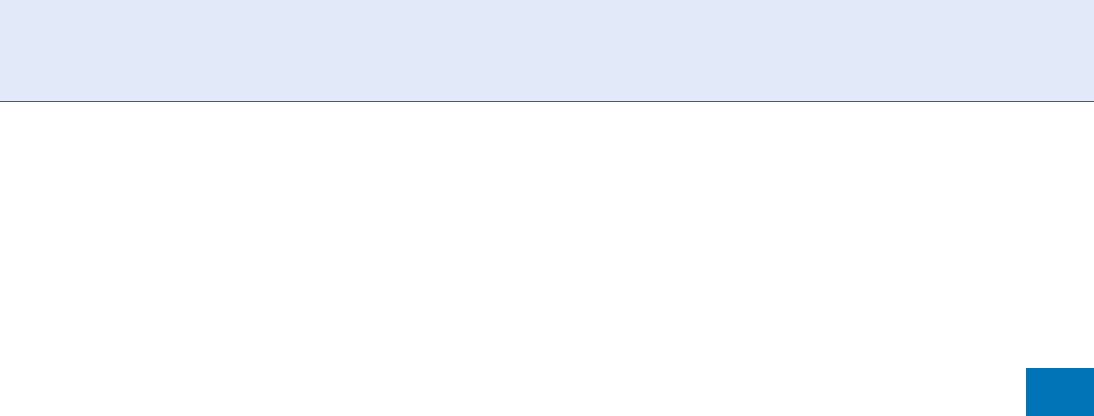
One reason that there may have been less effort
directed at these variations is the difficulty of obtaining
valid experimental data. For example, it would be
daunting to collect images of large numbers of subjects
before and after significant changes in weight. It is also
much more challenging to collect face images over a
period of many years than to collect image s from
different viewpoints, or with changes in lighting. The
government does collect photos of individuals over
long periods of time, for passports or drivers licenses,
for example, but privacy concerns prevent widespread
use of this data. As individuals post large collections of
personal photos on the internet there is a growing
opportunity to bu ild innovative new data sets of face
images, although by their nature, many of the ima-
ging conditions in these photos are uncontrolled
and unknown.
The complex set of factors that affect facial appear-
ance over time are discussed in [12]. In children, there
is significant change in face shape as they grow up. In
adults, there is less change in shape due to aging, and
more change in the appearance of skin due to exposure
to sunlight and the appearance of wrinkles [12] and
subsequent work describe experiments with a number
of recognition algorithms, including two commercial
systems, on data sets containing passport photos of
nearly 2,000 individuals, with a time lag between
photos ranging from 1–10 years. In a verification task
that asks whether two photos come from the same or
different people, performance is far below the levels
achieved using photos taken under controlled condi-
tions with little time lag [1]. It appears that there is
a sharp increase in the difficulty of recognition when
1 year passes between images, and that, at least for
adults, further passage of time, up to 10 years, creates
only small additional increases in difficulty. It is not
clear how much of these problems are due to aging,
and how much can be attributed to oth er changes in,
for example, weight or hair style that tend to occur
over time, or even to other factors such as artifacts
caused by the scanning of passport photos.
In addition to aging, a number of other sources of
variation have been mentioned in the literature, but
have not received much study. For example, a number
of researchers have noted that the presence or absence
of makeup on a face can affect the difficulty of recog-
nizing it, but there is little systematic work in this
area. Similarly, it is clear that significant changes in
weight can affect facial appearance, but there has been
little if any work in this area. Variations in hair style or
grooming can also have a considerable affect on ap-
pearance; partially for this reason most approaches to
face recognition focus on the inner part of the face, and
attempt to ignore the outer head and hair. However,
since the outer head and hair seem to be important in
human face recognition, it seems that understanding
hair appearance and its variations could be of potential
value in face recognition systems.
Conclusions
In summary, while most work on face recognition has
focused on settings in which there is little variation in a
face or in the viewing conditions, there is also a grow-
ing amount of work that addresses face variations.
In many cases, each source of variation has been
addressed with methods specific to that type of varia-
tion. For example, lighting variation has been attacked
using lighting insensitive image representations, while
pose methods often focus on the correspondence prob-
lem. Two exceptions are first, model-based methods,
such as those using morphable models, that extract a
3D model from an image, and then use computer
graphics to normalize its appearance and remove
face variations, and second, pattern recognition and
learning methods, such as Linear Discriminant Ana-
lysis, that can potentially characterize any specific
variation, provided there is appropriate training data.
Many face variations can cause significant degrada-
tion in performance in standard recognition methods.
While interesting progress has been made in develop-
ing recognition methods that account for these varia-
tions, these methods generally still have performance
that is substantially less than that can be achieved when
variations are controlled.
While many challenges remain, this article has
mentioned three in particular. First, there has been
little research aimed at developing methods suitable
for handling multiple simultaneous facial changes. For
example, it is not clear whether many of the methods
developed to handle lighting changes will be suitable
when there is also pose variation. Second, most work
has focused on situations in which the gallery images
are taken under uniform conditions. Surely, for exam-
ple, recognition will be more difficult when the gallery
contains a single image of each person taken with
different poses. Third, variations that occur over time
Face Variation
F
393
F