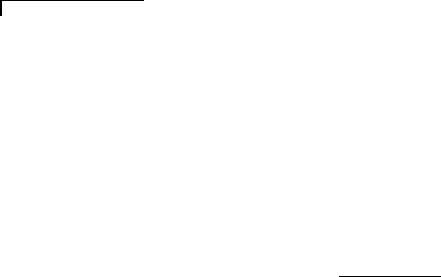
Statistics 205
∞ to 0. In other words, the probability, α, that the true variance is outside
of the sample variance precision interval increases as the precision interval
width decreases.
6.6.2 Establishing a Rejection Criterion
The relation between the probability of occurrence of a χ
2
value being less
than a specified χ
2
value can be utilized to ascertain whether or not effects
other than random ones are present in an experiment or process. This is
particularly relevant, for example, in establishing a rejection criterion for a
manufacturing process or in an experiment. If the sample’s χ
2
value exceeds
the value of χ
2
α
based upon the probability of occurrence P = 1 − α, it is
likely that systematic effects (biases) are present. In other words, the level of
significance α also can be used as a chance indicator of random effects. A low
value of α implies that there is very little chance that the noted difference
is due to random effects and, thus, that a systematic effect is the cause for
the discrepancy. In essence, a low value of α corresponds to a relatively high
value of χ
2
, which, of course, has little chance to occur randomly. It does,
however, have some chance to occur randomly, which leads to the possibility
of falsely identifying a random effect as being systematic, which is a Type II
error (see Section 6.8). For example, a batch sample yielding a low value of α
implies that the group from which it was drawn is suspect and probably (but
not definitely) should be rejected. A high value of α implies the opposite,
that the group probably (but not definitely) should be accepted.
Example Problem 6.5
Statement: This problem is adapted from [18]. A manufacturer of bearings has
compiled statistical information that shows the true variance in the diameter of “good”
bearings is 3.15 µm
2
. The manufacturer wishes to establish a batch rejection criterion
such that only small samples need to be taken and assessed to check whether or not
there is a flaw in the manufacturing process that day. The criterion states that when
a batch sample of 20 manufactured bearings has a sample variance > 5.00 µm
2
the
batch is to be rejected. This is because, most likely, there is a flaw in the manufacturing
process. What is the probability that a batch sample will be rejected even though the
true variance of the population from which it was drawn was within the tolerance limits
or, in other words, of making a Type II error?
Solution: From Equation 6.32,
χ
2
α
(ν) = νS
2
x
/σ
2
=
(20 − 1)(5.00)
(3.15)
= 30.16.
For this value of χ
2
and ν = 19, α
∼
=
0.05 using Table 6.5. So, there is approximately
a 5 % chance that the discrepancy is due to random effects (that a new batch will be
rejected even though its true variance is within the tolerance limits), or a 95 % chance
that it is not. Thus, the standard for rejection is good. That is, the manufacturer should
reject any sample that has S
2
x
> 5 µm
2
. In doing so, he risks only a 5 % chance of
falsely identifying a good batch as bad. If the χ
2
value equaled 11.7 instead, then there
would be a 90 % chance that the discrepancy is due to random effects.
Now what if the size of the batch sample was reduced to N = 10? For this case,
α = 0.0004. So, there is a 0.04 % chance that the discrepancy is due to random effects.