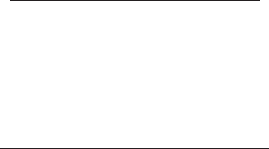
12.5 Testing for Significance 511
The point estimator is b
1
and the margin of error is t
α/2
. The confidence coefficient asso-s
b
1
ciated with this interval is 1 α, and t
α/2
is the t value providing an area of α/2 in the up-
per tail of a t distribution with n 2 degrees of freedom. For example, suppose that we
wanted to develop a 99% confidence interval estimate of β
1
for Armand’s Pizza Parlors.
From Table 2 of Appendix B we find that the t value corresponding to α .01 and
n 2 10 2 8 degrees of freedom is t
.005
3.355. Thus, the 99% confidence interval
estimate of β
1
is
or 3.05 to 6.95.
In using the t test for significance, the hypotheses tested were
At the α .01 level of significance, we can use the 99% confidence interval as an alterna-
tive for drawing the hypothesis testing conclusion for the Armand’s data. Because 0, the hy-
pothesized value of β
1
, is not included in the confidence interval (3.05 to 6.95), we can reject
H
0
and conclude that a significant statistical relationship exists between the size of the stu-
dent population and quarterly sales. In general, a confidence interval can be used to test any
two-sided hypothesis about β
1
. If the hypothesized value of β
1
is contained in the confi-
dence interval, do not reject H
0
. Otherwise, reject H
0
.
F Test
An F test, based on the F probability distribution, can also be used to test for significance
in regression. With only one independent variable, the F test will provide the same conclu-
sion as the t test; that is, if the t test indicates β
1
0 and hence a significant relationship,
the F test will also indicate a significant relationship. But with more than one independent
variable, only the F test can be used to test for an overall significant relationship.
The logic behind the use of the F test for determining whether the regression relation-
ship is statistically significant is based on the development of two independent estimates of
σ
2
. We explained how MSE provides an estimate of σ
2
. If the null hypothesis H
0
: β
1
0 is
true, the sum of squares due to regression, SSR, divided by its degrees of freedom provides
another independent estimate of σ
2
. This estimate is called the mean square due to regres-
sion, or simply the mean square regression, and is denoted MSR. In general,
For the models we consider in this text, the regression degrees of freedom is always
equal to the number of independent variables in the model:
(12.20)
Because we consider only regression models with one independent variable in this chapter, we
have MSR SSR/1 SSR. Hence, for Armand’s Pizza Parlors, MSR SSR 14,200.
If the null hypothesis (H
0
: β
1
0) is true, MSR and MSE are two independent estimates
of σ
2
and the sampling distribution of MSR/MSE follows an F distribution with numerator
MSR
SSR
Number of independent variables
MSR
SSR
Regression degrees of freedom
H
0
:
H
a
:
β
1
0
β
1
0
b
1
t
α/2
s
b
1
5
3.355(.5803) 5
1.95
CH012.qxd 8/16/10 6:59 PM Page 511
Copyright 2010 Cengage Learning. All Rights Reserved. May not be copied, scanned, or duplicated, in whole or in part. Due to electronic rights, some third party content may be suppressed from the eBook and/or eChapter(s).
Editorial review has deemed that any suppressed content does not materially affect the overall learning experience. Cengage Learning reserves the right to remove additional content at any time if subsequent rights restrictions require it.