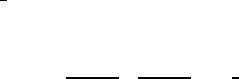
358 THE CONFORMAL MODEL: OPERATIONAL EUCLIDEAN GEOMETRY CHAPTER 13
vectors out of them: the vectors (e +
-
e) and (
-
e − e) are both null without being zero, as you
can check easily by squaring them. In this construction, the representational space has an
orthonormal basis consisting of (n + 1) positive dimensions (with basis vectors squaring
to +1) and 1 negative dimension (with basis vector squaring to −1). We therefore denote
it by
R
n+1,1
. Such a space is called a Minkowski space in physics, where it has been well
studied to represent space-time in relativity; the negative dimension is then employed
to represent time. We will find a geometrically more natural basis than e and
-
e for the
conformal model, but the representational space is still the metric space
R
n+1,1
.
This (n + 2)-dimensional space requires n + 2 coefficients to specify a vector. We should
require only n coefficients to specify a point in Euclidean space, so there is more in this
space than we appear to need. Since points are represented by null vectors, their repre-
sentatives must obey p · p = 0, and this is a condition on their coefficients that removes
onedegreeoffreedom,leavingn + 1. The remaining degree of freedom we use to denote
the weight of the point, as in the homogeneous model. In that model, we retrieved the
weight as e
−1
0
· p, since it was the coefficient of e
0
; in the present model we extract it by
the operation −∞ · p. The relationship of inner product to distance of (13.1) should of
course be defined on properly normalized points, since it is independent of the weight,
so in that formula we should divide p by its weight, and q as well. We introduce an extra
scaling factor of −
1
2
for convenience later on, and actually define the inner product of
vectors representing points through
p
−∞ · p
·
q
−∞ · q
≡−
1
2
d
2
E
(P, Q). (13.2)
(The two minus signs on the left of course cancel each other, but we would like to get you
used to viewing −∞ · p as a weight-computing expression.) Equation (13.2) is in fact the
definition of the representational model; all the rest of the correspondence between ele-
ments of its geometric algebra and Euclidean geometry follows from it without additional
assumptions.
The model thus constructed was called the conformal model in the literature, for the
mathematical reason that it is capable of representing conformal transformations by ver-
sors. We will demonstrate that in Chapter 16, but prefer to focus on its use for Euclidean
transformations first. We will show that it is an operational model for Euclidean geom-
etry in the double sense that Euclidean transformations are represented as structure-
preserving operators, which are moreover easy to put to operational use. In view of that,
we would prefer to call it the operational model for Euclidean computations. This is too
much of a mouthful, so we might as well conform to the term conformal model, despite
its arcane origins.
Even though the conformal model hit computer science only a few years ago, when it was
introduced by Hestenes et al. in 1999 [31], we are beginning to find that we could have had
the pleasure of its use all along. Considerable elements of it are found in much older work,
and it appears to have been reinvented several times. We do not know the whole story yet,