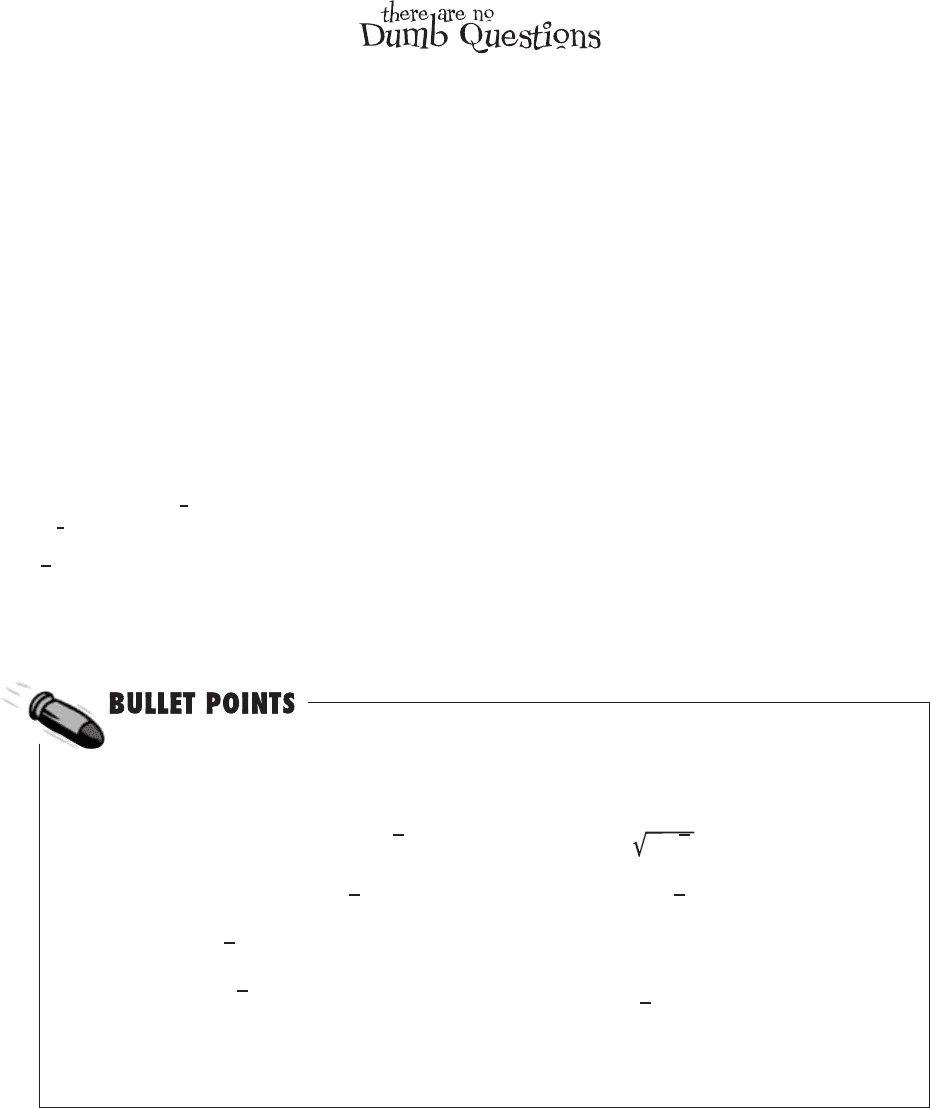
you are here 4 485
estimating populations and samples
Q:
Do I need to use any continuity
corrections with the central limit
theorem?
A: Good question, but no you don’t.
You use the central limit theorem to find
probabilities associated with the sample
mean rather than the values in the sample,
which means you don’t need to make any
sort of continuity correction.
Q:
Is there a relationship between
point estimators and sampling
distributions?
A: Yes, there is.
Let’s start with the mean. The point estimator
for the population mean is x, which means
that μ = x. Now, if we look at the expectation
for the sampling distribution of means, we
get E(X) = μ. The expectation of all the
sample means is given by μ, and we can
estimate μ with the sample mean.
Similarly, the point estimator for the
population proportion is p
s
, the sample
proportion, which means that p = p
s
. If
we take the expectation of all the sample
proportions, we get E(P
s
) = p. The
expectation of all the sample proportions is
given by p, and we can estimate p with the
sample proportion.
We’re not going to prove it, but we get a
similar result for the variance. We have
σ
2
= s
2
, and E(S
2
) = σ
2
.
Q:
So is that a coincidence?
A: No, it’s not. The estimators are chosen
so that the expectation of a large number
of samples, all of size n and taken in the
same way, is equal to the true value of
the population parameter. We call these
estimators unbiased if this holds true.
An unbiased estimator is likely to be
accurate because on average across all
possible samples, it’s expected to be the
value of the true population parameter.
Q:
How does standard error come into
this?
A: The best unbiased estimator for a
population parameter is generally one with
the smallest variance. In other words, it’s the
one with the smallest standard error.
The sampling distribution of means is what
you get if you consider all possible samples of
size n taken from the same population and form
a distribution out of their means. We use X to
represent the sample mean random variable.
The expectation and variance of X are defined as
E(X) = μ
Var(X) = σ
2
/n
where μ and σ
2
are the mean and variance of the
population.
The standard error of the mean is the standard
deviation of this distribution. It’s given by
Var(X)
If X ~ N(μ, σ
2
), then X ~ N(μ, σ
2
/n).
The central limit theorem says that if n is large
and X doesn’t follow a normal distribution, then
X ~ N(μ, σ
2
/n)
^