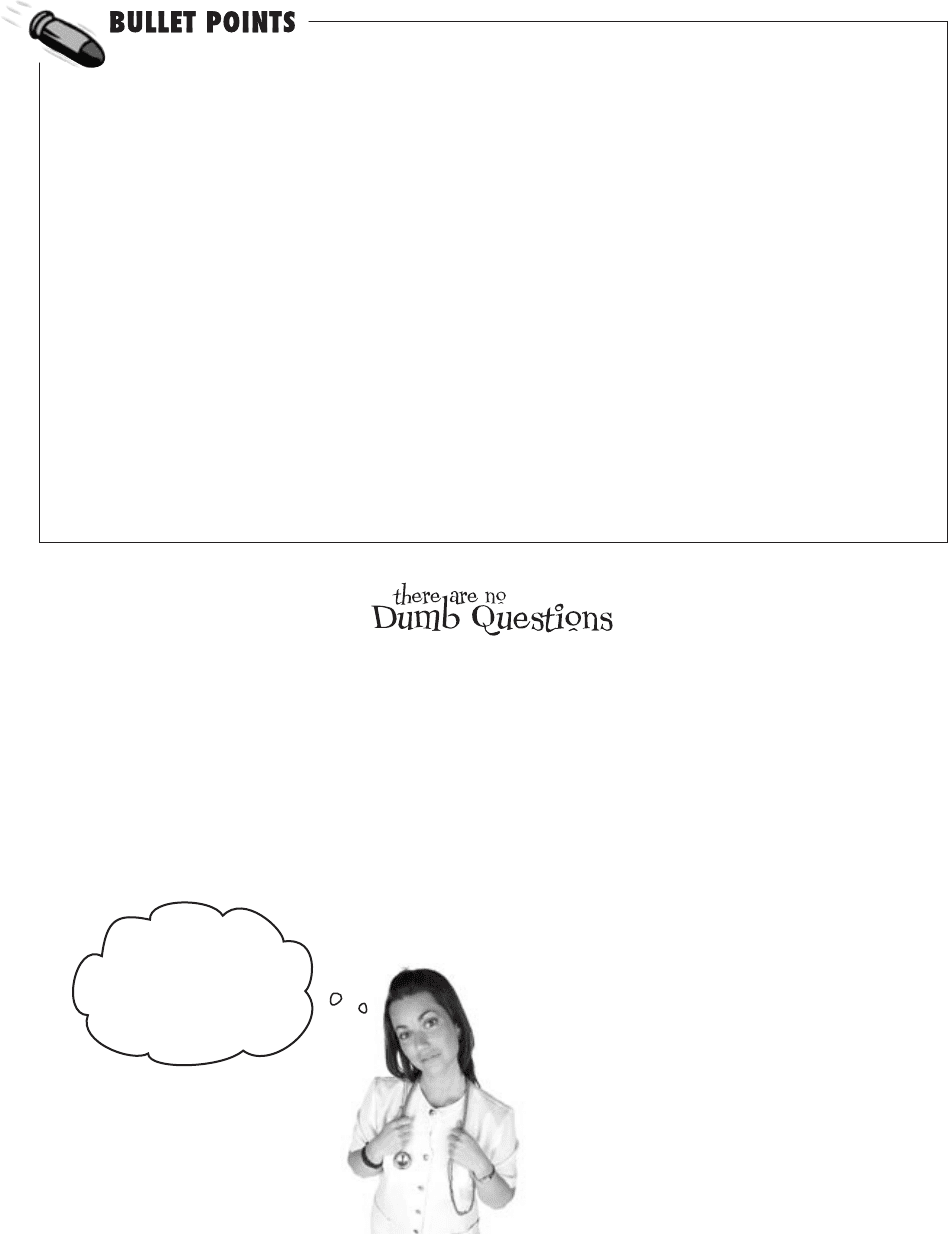
you are here 4 539
using hypothesis tests
Q:
What significance level should I normally test at?
A: It all depends how strong you want the evidence to be before
you reject the null hypothesis. The stronger you want the evidence to
be, the lower your significance level needs to be.
The most common significance level is 5%, although you sometimes
see tests at the 1% level. Testing at the 1% means that you require
stronger evidence than if you test at the 5% level.
Q:
Does the significance level have anything in common with
the level of confidence for confidence intervals?
A: Yes, they have0 a lot in common. When you construct a
confidence interval for a population parameter, you want to have
a certain degree of confidence that the population parameter lies
between two limits. As an example, if you have a 95% level of
confidence, this means that the probability that the population
parameter lies between the two limits is 0.95.
The level of significance reflects the probability that values will lie
outside a certain limit. As an example, a significance level of 5%
means that your critical region must have a probability of 0.05.
I still have doubts.
I wonder what would
happen if I took a
larger sample...
In a hypothesis test, you take a claim and test it
against statistical evidence.
The claim that you’re testing is called the null
hypothesis test. It’s represented as H
0
, and it’s the
claim that’s accepted unless there’s strong statistical
evidence against it.
The alternate hypothesis is the claim we’ll accept
if there’s strong enough evidence against H
0
. It’s
represented by H
1
.
The test statistic is the statistic you use to test your
hypothesis. It’s the statistic that’s most relevant to
the test. You choose the test statistic by assuming
that H
0
is true.
The significance level is represented by α. It’s a way
of saying how unlikely you want your results to be
before you’ll reject H
0
.
The critical region is the set of values that presents
the most extreme evidence against the null
hypothesis test. You choose your critical region by
considering the significance level and how many tails
you need to use.
A one-tailed test is when your critical region lies
in either the upper or the lower tail of the data. A
two-tailed test is when it’s split over both ends.
You choose your tail by looking at your alternate
hypothesis.
A p-value is the probability of getting the result
of your sample, or a result more extreme in the
direction of your critical region.
If the p-value lies in the critical region, you have
sufficient reason to reject your null hypothesis. If
your p-value lies outside your critical region, you
have insufficient evidence.